A HYBRID Event with Both IN-PERSON and VIRTUAL Attendance Options
Conference Theme: Field Implementation of PLF
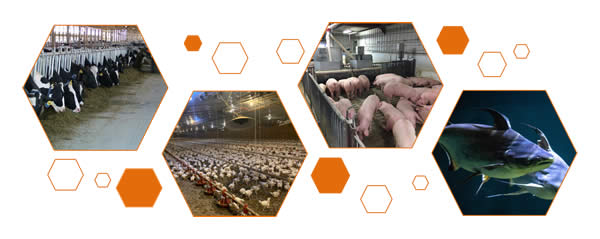
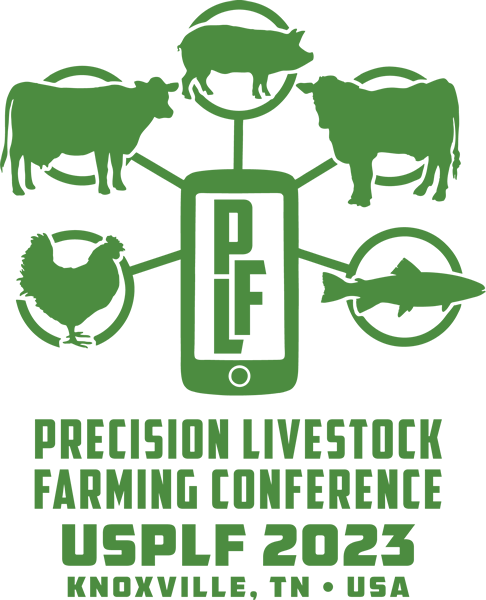
May 21 – 24, 2023
Knoxville, Tennessee
usplf2023.utk.edu
Exploring the Insights of USPLF 2023: Access Conference Publications
Thank you for being part of the success of USPLF 2023! The conference has concluded, but the knowledge shared and discussed during this event lives on through the insightful publications presented by our esteemed speakers.
Discover the wealth of information and research shared at USPLF 2023 by accessing the conference papers below. Dive into the groundbreaking ideas, innovative discoveries, and thought-provoking insights offered by our community of researchers and experts.
Click on the links provided to explore the wealth of knowledge presented during the conference. Should you have any questions or require further information, feel free to reach out to us.
We extend our gratitude to all participants, presenters, and contributors for making USPLF 2023 a resounding success. Let’s continue the journey of learning and discovery together.”
- SESSION 1
Social Dimensions and Impacts - SESSION 2
Vision - SESSION 3
Data Modeling - SESSION 4
Disease Monitoring - SESSION 5
Precision Livestock Farming Systems - SESSION 6
Weight and Dimensions - SESSION 7
Heat Stress - SESSION 8
Broiler Welfare and Applications - SESSION 9
Identification - SESSION 10
Beef Cattle Applications - SESSION 11
Wearables and Behavior - SESSION 12
Ventilation, Indoor Air Quality, and Emissions - SESSION 13
Applications for Laying Hens – Aviaries - SESSION 14
Applications and Sensors in Swine Production - POSTERS
- ABSTRACTS
Abstract. As precision livestock farming (PLF) technologies emerge, it is important to consider their social and ethical dimensions. Reviews of PLF have highlighted the importance of considering ethical issues related to privacy, security, and welfare. However, little attention has been paid to ethical issues related to transparency regarding these technologies. This paper proposes a framework for developing responsible transparency in the context of PLF. It examines the kinds of information that could be ethically important to disclose about these technologies, the different audiences that might care about this information, the challenges involved in achieving transparency for these audiences, and some promising strategies for addressing these challenges. For example, with respect to the information to be disclosed, efforts to foster transparency could focus on: (1) information about the goals and priorities of those developing PLF systems; (2) details about how the systems operate; (3) information about implicit values that could be embedded in the systems; and/or (4) characteristics of the machine learning algorithms often incorporated into these systems. In many cases, this information is likely to be difficult to obtain or communicate meaningfully to relevant audiences (e.g., farmers, consumers, industry, and/or regulators). Some of the potential steps for addressing these challenges include fostering collaborations between the developers and users of PLF systems, developing techniques for identifying and disclosing important forms of information, and pursuing forms of PLF that can be responsibly employed with less transparency. Given the complexity of transparency and its ethical and practical importance, a framework for developing and evaluating transparency will be an important element of ongoing PLF research.
Abstract. Precision Livestock Farming (PLF) technologies offer a way to improve production and labor efficiency while also addressing welfare concerns that consumers express regarding how poultry is grown. There is an extreme lack of knowledge on the current perceptions and need of PLF within the U.S. poultry academia. The objective of this study was to design a survey to understand the perceptions and needs of PLF by academia. An online survey was designed via Qualtrics consisting of 25 questions. The survey was distributed via email to 276 poultry researchers in the U.S. acquired via search on university websites. The survey was made available for one month and yielded 68 total responses (N=68). The results were exported from Qualtrics and analyzed using SPSS. Respondents were separated into groups based on job type (extension specialist, researcher, both) and research type (animal science, engineer, vet). Results were analyzed using a chi-square test, frequency analysis, and the Monte Carlo feature to account for the relatively small sample size. Amongst researchers, results from this study show that 92% of researchers agree that cost influences a grower’s decision to implement PLF technology and 86% of researchers agree usability influences a farmer’s decision to implement. The results also show most poultry researchers are familiar with the term PLF (92%), however, only 55% of respondents have or currently use PLF technologies. Researchers also agree that PLF will be very important in improving poultry production (97%), and health and welfare (91%) when compared by research type there were no significant differences (p=0.36) found amongst groups regarding health and welfare, however, it was found that engineers agree more than animal scientists that PLF will be important to improve future poultry production with 61% of engineers strongly agreeing comparing to only 34% from animal scientists (p=0.02). Significant differences were also found when the researchers who already use PLF in which 57% strongly agreed that PLF will allow them to expand their research compared to researchers who do not use PLF in which only 13.3% strongly agreed (p=0.01). It can be concluded that poultry researchers have realized the importance of PLF’s role in poultry production, but applications of PLF technologies in research remain to grow. In addition, perceptions on PLF vary among researchers with different backgrounds.
Keywords: perceptions, precision livestock farming, health and welfare, production, academia
Abstract. To remain sustainable, the livestock industries must demonstrate commitment to addressing animal welfare concerns from the public. Researchers have proposed precision dairy technologies (PDTs) as a potential solution to ameliorate concerns from the public. The aims of this review were to identify methods used by researchers in the investigation of public perceptions of PDTs and describe the current knowledge of public perceptions. Three online databases (Google Scholar, PubMed, and Web of Science Core Collection) were used to search for scientific articles that investigated public concerns and attitudes toward the use of technology in the context of dairy farming. A total of 453 publications were screened; two publications fit all inclusion criteria and were included in this review. Both focused on studies conducted among the European public, with one using publicly distributed surveys and another using focus groups. Investigated topics included the state of public perceptions related to PDTs, as well as desires for using technology on dairy farms. Public concerns related to three major concepts: 1) industrialization in agriculture, 2) transparency of dairy products, and 3) animal welfare. Knowing more about how the public views technology use as well as how to best engage society in conversations about technology use on farms is integral to improving the social sustainability of the dairy industry.
Keywords: animal welfare, precision dairy technology, public perception, social science
Abstract. This study used Q methodology to analyze perceptions of precision livestock farming (PLF) technology held by stakeholders directly or indirectly involved in the swine industry. We identified three distinct points of view: PLF improves farm management, animal welfare, and laborer work conditions; PLF does not solve swine industry problems; PLF has limitations and could lead to data ownership conflict. Stakeholders with in-depth knowledge of PLF technology demonstrated elevated levels of optimism about it, whereas those with a basic understanding were skeptical of PLF claims. Despite holding different PLF views, all stakeholders agreed on the significance of training to enhance PLF usefulness perceptions and its eventual adoption. In conclusion, we believe the study’s results hold promise for helping the swine industry stakeholders make better-informed decisions about the PLF technology implementation.
Keywords: precision livestock farming, Q-methodology, stakeholder, swine industry
Abstract. There is some disagreement about whether animal welfare will be helped or hindered by Precision Livestock Farming technologies in the small but important and growing literature on the ethics of PLF. This paper will argue that these disagreements are sometimes based not on disagreements about current or future empirical facts, but rather different concepts of “welfare,” some of which are in tension with one another. At the same time, the discourse around current and future PLF technologies also promises an increase in transparency and traceability of animals through their lives and food production by farmers, governments, and consumers. This paper argues that this transparency and traceability also allows for a partial resolution of the above disagreements around animal welfare, or at least a step forward in the discourse around it. It can do this by creating complex pictures of welfare down to the level of individual animals that can then be used to examine tradeoffs and to inform activism and preference expression by everyone in the food system. We will look at objections to this picture on various grounds, but ultimately this paper argues that the information provided by PLF technologies is useful not only to farmers but to people at every stage of the food system. This information can facilitate discourse and public deliberation around animal welfare, and allow people to better understand and act on the plural values we have toward our relationships with and uses of non-human animals.
Abstract. Artificial intelligence (AI) has become one of the most important technologies in different fields of science during the last decades. However, widespread access to AI algorithms and solutions is yet to become a reality for non-expert users. Open-source repositories host several deep learning algorithms used in AI, but they still require users to have programming experience to benefit from them. Tools designed using non-coding policies would positively impact communities of non-experts in many scientific domains, create new means for teaching in K-12 and higher education, and benefit underrepresented groups in minority serving institutions. In this project we developed an open-source software to democratize the access to deep learning algorithms. Such software will be used to perform image classification by training new and customized deep leaning algorithms on datasets provided by the user. An easy-to-use user interface was developed to allow users with no previous programming experience to train their own image classification neural networks and use such networks to perform predictions on newly chosen images. The software suite was implemented in Python using TensorFlow and Keras deep learning libraries. Developing easy-to-use open-source tools for Image Classification will help a large number of individuals who aim to learn and experiment with AI technology. Our goal is to create more accessible user interfaces so that coding ability is not a barrier.
Keywords: artificial intelligence, democratization, image analysis, machine learning
Abstract. Precision livestock management (PLM) uses innovative sensing tools, data analytics, and modeling techniques to support decision-making and advance animal production monitoring. However, there is limited effort focused on PLM applications specific to the beef cattle industry in the U.S. Unlike other species, beef production situates uniquely due to its extensive housing environment, with most operations being extensively outdoors. This major difference poses a significant challenge to the beef industry’s development and adoption of modern PLM technologies. In addition, animal practitioners continue to question the application, commercial success, implementation possibilities, and economic benefits of PLM tools used in different beef cattle management systems. Thus, whether these techniques can bring new opportunities for PLM research and production in the beef industry – particularly the U.S. feedlot sector – requires further evaluation. A survey questionnaire was developed and distributed to Nebraska and adjacent states’ commercial feedlot producers on their knowledge and needs regarding PLM technologies. Twelve questions captured producer feedback on 1) their PLM knowledge level; 2) their willingness, acceptance level, and strategy in adopting such technologies; 3) their biggest concerns in the daily feedlot operations; 4) PLM ideas/technologies that interest them; 5) their comfort level (dollar-for-dollar) on investing in PLM technologies, and 6) their role and operation size. The survey was well received by feedlot producers, with 78 completed surveys received. Survey results highlighted the significance, challenges, and opportunities of developing and evaluating PLM tools for the beef feedlot industry and covered more than 574,000 feedlot cattle managed. The results of the survey are summarized in this conference paper.
Keywords: beef cattle, extension, need assessment, questionnaire, survey
Abstract. For over 100 years, Extension educators have used field demonstrations to provide farmers with the needed information to consider the implementation of new practices. Demonstration facilities provide a unique opportunity for hands-on learning, troubleshooting, and applied research opportunities. University research stations and cooperating private farms have proven to be excellent settings for field demonstrations of animal management, new crop varieties, herbicides, pesticides and tillage practices. Specialized equipment, data privacy, ownership concerns, and overall investment level have been obstacles to initiating full-scale Precision Livestock Farming demonstrations on both university and private farms. To address this challenge, Tennessee AgResearch is equipping selected research and education centers to support the applied research and demonstration of beef, broiler and dairy Precision Livestock Farming systems. This paper reports on the establishment of full-scale beef and broiler Precision Livestock Farming units at the Middle Tennessee AgResearch and Education Center in central Tennessee, and at a full-scale dairy operation at the Little River Animal and Environment Unit in eastern Tennessee. Details about the establishment of pasture-based beef facilities, four commercial broiler houses, and two robotic milking systems that will be used to support Precision Livestock Farming system demonstrations are provided. These new and updated Precision Livestock Farming research facilities will provide producers with the opportunity to see the potential impact of Precision Livestock Farming across a variety of animal species and management styles. Additionally, these facilities will allow training of producers, industry members, Extension personnel, teachers, and researchers.
Keywords: precision livestock farming, full-scale, beef, dairy, broiler
Abstract. Body trait measurements (e.g., body weight) are routinely performed and provide critical information for producers to assess growth, reproductive performance, and marketing window for individual beef cattle in extensive ranches. However, most of the body trait information is obtained via visual assessment conducted by experienced workers, which has a high rate of inaccuracy. This study aimed to estimate the live body weight of individual beef heifers via three-dimensional (3D) sensing, point-cloud reconstruction, and machine learning regression. 67 beef yearling heifers weighing between 282.13 and 439.98 kg were used for data collection. Top-view RGB-depth videos were recorded when individual heifer walked through by using a 3D sensing camera. Selected RGB-depth images were used to reconstruct point clouds, in which backgrounds were removed. Cattle point clouds were preprocessed to extract 2D and 3D features. Eight machine learning regression models were developed and assessed to determine the optimal model for estimating body weights. Results show that middle slices along x- and y-axis provide good cattle’s back features and support estimation purposes. Using the Adaptive Bosting model, the best R2 and mean absolute errors for liveweight regression were 0.934 and 5.632 kg, respectively. The developed pipeline, including preprocessing of point clouds, point clouds feature extraction, and machine learning model prediction, took 3.670±0.013 seconds per heifer point cloud for weight regression. The developed techniques provide supportive tools for precision beef cattle management.
Keywords: artificial intelligence, body weight, computer vision, high-throughput phenotyping
Abstract. Research has shown that posture records can be used to assess the health, welfare, and estrus status of sows and gilts which are critical for the commercial swine industry. Individual animals can be monitored in real-time using imagery sensors and accelerometers to quantify their behavior patterns in a large herd. In this study, a rear-view robotic imaging system, consisting of a LiDAR camera, edge-computing unit, and robotic platform, was developed to monitor the behavior of sows/gilts housed in gestation stalls. Collected images were used to classify the four types of postures, i.e., STA: standing, SIT: sitting, SL: sternal lying, and LL: lateral lying, using convolutional neural networks (CNNs). In this study, eight sows were continuously monitored at a 5-minute interval for 30 days. All sows were managed and their estrus checks were conducted according to standard farm operation protocols. Performance between four CNN models (VGG16, MobileNet, Xception, and DenseNet121) was compared. Results show VGG16 had significantly lower validation accuracy (99.8%, p<0.05) compared to MobileNet, Xception, and Densnet121 (99.91% ± 0.04%). The Xception model yielded significantly higher test accuracy (98.25%). The MobileNet model required significantly less time to process each image (t = 210ms). Daily activity level (calculated as the portion of STA and SIT postures) and semi-idle level (calculated as the portion of SL posture) on the day of onset of estrus were significantly higher than those on the previous days. Daily idle level (the portion of LL posture) decreased significantly on the day of the onset of estrus. In addition, the absolute change of daily idle level was significantly higher than that of daily activity and semi-idle level (p<0.05), suggesting that daily idle level could be a more reliable indicator than daily activity level for identifying the onset of estrus events. In this research, no distinct behavior pattern was observed around the expected return estrus. The study concluded that posture patterns derived from the robotic imaging system could help detect sows’ first onset of estrus but might not help detect return estrus.
Keywords: swine reproduction; estrus detection; robotic imaging system; 3D camera; digital agriculture
Abstract. Locomotion of piglets is a critical indicator of their growth, health, and welfare status; thus it is of utmost importance to automate the analysis of piglet locomotion, particularly during the early lactation periods. An intersection over unit- (IOU-) and contour-based tracking method is proposed to automate the locomotion analysis for piglets in farrowing pens. In the first step, an anchor-free deep learning network is employed in amodal instance segmentation of individual piglets. Then a novel attention graph convolution-based structure is used to distil element-wise features within the detected piglets. The distilled features are further encoded by a graph convolutional network. In the output features, pixels selected by a selection strategy derive features for a real-value pixel using 4-point nearest neighbor bilinear interpolation. Thus, a higherresolution segmentation is predicted in a coarse-to-fine fashion. In the second step, a regional matching strategy is adopted synchronously for initial tracking. The Hungarian algorithm is then used to optimize initial tracking trajectories determined by contour features and IOUs. In the experiment, our method produced crisp amodal instance segmentation, whilst also achieving favorable tracking performance under occlusion. The tracking results demonstrated an IDF1 score of 95.7% and an MOTA of 97.1% in short video clips. With the high-accuracy occlusion-resistant segmentation and tracking results, our computer vision-based piglet tracking method may aid automated piglet locomotion and behavior analysis.
Keywords: animal welfare, occlusion, multiple object tracking, deep learning
Abstract. Calves’ health and welfare are critical on any dairy farm because they are the basis for remounting. Therefore, monitoring calf health to raise well-developed calves is crucial to successful calf management. Due to advancing digitalization, automatic monitoring systems allow for conclusions about possible animal diseases based on various parameters, like activity. Thus, these systems are widely used in livestock farming. Progress can also be observed in calf husbandry, even if the number of systems is still small. This study aims to validate the suitability of a passive infrared sensor system used in individual calf housing to detect the activity levels of calves. The passive infrared detector (PID) was attached to three separate pens to assess the accuracy under practical conditions. We installed a camera system as a reference method for activity monitoring to record the three pens during the six-month observation period. A trained person evaluated the video footage, extracting the calves’ behavior using an ethogram. The video-based behavior assessment was compared to the sensor-detected activity levels for three focus calves (72 hours per calf). The results showed that the PID could detect general changes in activity. In addition, the circadian rhythm of the calves could be reproduced. A deviation in activity levels occurred when a calf was lying down, which may have been caused by the calf’s coat.
Keywords: calf husbandry, animal welfare, livestock management
Abstract. The effects of reduced growth rate on welfare and behavior in the same broiler strain have never been scientifically studied. This study evaluated the welfare and behaviors of Cobb 700 broilers as affected by growth rate (GR) and stocking density (SD). Slow-growing (GR < 50 g/day) and medium-growing (GR = 50 – 60 g/day) broilers were produced via providing 60% and 80% of the feed intake recommended in the Cobb 700 production manual for standard broilers (GR > 60 g/day). The broilers at all three GRs were reared at two stocking densities of 30 and 40 kg/m2. Broiler feather coverage were evaluated when birds reached 1, 2 and 3 kg of body weights. Activity index was determined by top cameras and image processing, and feeding hours were recorded using the radio-frequency identification (RFID) systems. It held 45 days for standard, 52 days for medium-growing, and 62 days for slow-growing broilers to reach 3 kg market body weight. Feed conversion ratios (FCR, kg feed per kg weight gain) were 1.80 for slow-growing, 1.67 for medium-growing and 1.57 for standard broilers. Slow-growing broilers were more active and had better feather coverage, yet standard broilers showed best production performance. In conclusion, lowered GR and SD may improve certain broiler welfare conditions at a high expense of compromised production performance and prolonged production cycle.
Keywords: broiler, growth rate, stocking density, welfare, behavior, PLF technology
Abstract. Monitoring the drinking behavior of animals is of great importance for livestock farming. Measuring drinking time is labor demanding and thus, it is still a challenge in most livestock production systems. Computer vision technology using a low-cost camera system can be useful in overcoming this issue. Therefore, the aim of this research was to develop a computer vision system for monitoring beef cattle drinking behavior. A data acquisition system, including an RGB-D camera and an ultrasonic sensor was developed to record the beef cattle drinking actions. We developed an algorithm for tracking the beef cattle key body parts, such as headear-neck position, using a state-of-the-art deep learning architecture DeepLabCut. The extracted key points were analyzed using a Long Short-Term Memory (LSTM) model to classify drinking and non-drinking periods. A total of 70 videos were used to train and test the model and 8 videos were used for validating purposes. During the testing, the model achieved 97.35% accuracy. The results of this study will guide us to meet the immediate needs and expand farmers’ capability in monitoring animal health and well-being.
Keywords: animal behavior, drinking time, computer vision, precision livestock farming
Abstract. Shoulder lesion in sows is a common welfare concern with prevalence ranging from 4.6 to 50% occurrence. They represent not only a welfare concern but also have an economic impact due to labor needed for treatment and medication, in addition to the premature culling of affected sows. The objective of this study is to evaluate the use of computer vision techniques in detecting and determining the size of shoulder lesions. A time-of-flight (Microsoft Kinect V2) camera was used to capture the top-down depth and RGB images of sows in farrowing crates. This camera has an RGB image resolution of 1920 ×1080. To ensure the best view of the lesions, images were selected with sows lying on their right and left sides with all legs extended. A total of 320 RGB images from 28 sows with lesions at various stages of development were identified and annotated. Two deep learning models (YOLOv5 and FRCNN) pre-trained with the COCO dataset were implemented. The model was tested on 90 images (70 images with lesions, and 20 images without lesions) outside the training set. YOLOv5 was able to detect lesions better than FRCNN with an mAP@0.5 of 0.921. Next, image processing techniques like binarization were used to estimate lesion sizes in measurable units. Lesion size was determined using hand-selected ROI with ImageJ software. The estimated size matched the hand-determined size with an R2 = 0.91.
Keywords: shoulder lesions, ulcers, sows, deep learning, YOLO
Abstract. Fetch cows, dairy cattle that do not voluntarily enter automated milking systems (AMS), increase labor costs and decrease efficiency. The objective of this study was to describe fetch cows based on fetch lists and herdmanagement software data. This study was conducted on a commercial dairy (n = 528 cows) using 8 AMS from October 1, 2021 to May 10, 2022. Data were collected via PCDART, DairyComp, and a proprietary interface. Two fetch categories, EverFetch (EF) and NeverFetch (NF), and three status groups, Yes (on fetch list and fetched), No (on fetch list and not fetched), and NA (not on list), were created. The impact of health events (no event, reproduction, general health, veterinary checks, and miscellaneous) and parity (1, 2, and 3+ lactations) on fetch category and status were analyzed using the GLIMMIX procedure of SAS 9.4 (P ≤ 0.05). Cows that experienced general health events compared to no events were more likely (odds ratio (OR), confidence interval (CI) = 3.8, 2.2 to 6.4; P < 0.01) to be EF. Compared to 2nd, 1st lactation cows were more likely (OR, CI = 2.1, 1.2 to 3.4; P < 0.02) to be EF. Second and 3+ lactation cows were more likely (OR, CI = 1.7, 1.0 to 2.8; 1.26 to 3.5, respectively, P < 0.01) to be fetched than 1st lactation. Cows that experienced a health event and younger cows had a greater chance of being on the fetch list. However, 2 and 3+ lactations were more likely to be fetched.
Keywords: Farm management software, Automated milking systems, Farmer usefulness
Abstract. Feed efficiency (FE) in milking cows is squarely linked to profitability due to its positive correlation with income over feed cost (IOFC). In turn, IOFC is an indicator that ties nutrition to profitability of dairy operations. However, monitoring these key performance indicators (KPI) on the farm continuously and consistently can be difficult as it necessitates merging data from various sources. Thereby, data integration through the application of data engineering techniques becomes mandatory to achieve it. We have been successfully developing a robust system that integrates data from disparate on and off-farm data sources and provides FE, IOFC, and other KPIs on a continuous basis. Thus, the objective of this work is to describe the process involved in developing such a system and to demonstrate how it helps farmers to make decisions in a more timely manner. The calculations require integration of data from: 1) farm’s feeding system; 2) the milking parlor system; and 3) milking processor report to correct the milk yield to a standard value of energy and milk prices. All data collected is parsed from their native file formats and loaded into a data warehouse. The FE, IOFC, and other KPIs are made available in a user-friendly report to the farmer. Thus, this work demonstrates that it is possible to constantly integrate disparate sources of data and add value to data in dairy farms, helping farmers to get improved decisions. Furthermore, it demonstrates that the active involvement of the farmer in the process makes its results more robust and meaningful.
Keywords: data engineering, decision-making, feed intake, income over feed cost, milk yield
Abstract. Among technologies used for animal high-throughput phenotyping, computer vision generates large amounts of unstructured data. The development of automated data pipelines using scalable solutions such as cloud computing, can be an effective strategy to generate animal-level information in real-time. The main objective of this study was to develop a cloud computing-based system to automate phenotype extraction in dairy farms using computer vision systems. In this study we used animal identification and body condition score (BCS) as case studies to demonstrate the automation pipelines for data collection and analyses. The proposed system outputs a predicted BCS for each cow individually each day, using depth and infrared images captured from cameras installed at the exit of the milking parlor and uploaded to the cloud. The entire pipeline is performed on the cloud and consists of four different steps: (1) determine good quality images; (2) remove pixels that do not belong to the cow’s body; (3) identify the cow based on coat color patterns; (4) and assess BCS using depth images. The neural networks designed to identify individual cows and score BCS achieved accuracies of 94% and 71%, respectively, on a group of 59 cows, with a mean absolute error of 0.20 for BCS. From a total of 52,247 snapshots, 32,655 were discarded in step 1, and the remaining 19,592 were evaluated. All predicted BCS values and the associated animal identification (ID) are automatically stored in a relational database once a new snapshot is captured and sent to the cloud. The main contribution of this work is the development of a scalable cloud-based framework for large-scale individual phenotyping in livestock systems using computer vision techniques.
Keywords: cloud computing, computer vision, dairy cattle, body condition score
Abstract. Precision Dairy Farming is a term that describes the benefit of using digital technologies to measure physiological, behavioral, and production indicators for individual animals. SomaDetect™ has developed a series of algorithms to monitor individual cow performance and health at every milking as a major advancement for Precision Dairy Farming (PDF). The methods used include a light-scattering sensor, cloudbased IoT infrastructure, and data processing algorithms to develop actionable shortlists that are delivered via software to the farm users. The system has been tested across two sizes of farms: small (<200-cow herd) and large (>3000-cow herd), and across a four-month period to test for temporal stability. In this paper, the approach used is described in detail and provides examples to show how the data can be used to derive economic value for dairy farms via actionable shortlists. Results from the various studies presented demonstrate that the shortlists provided by the SomaDetect ecosystem facilitate data-driven, practical, strategic management decision changes. These management interventions drive farm Key Performance Indicators (KPIs) and enable several valuable impacts such as reducing the Bulk Tank Somatic Cell Count (BTSCC), enabling Selective Dry Cow Therapy (SDCT), and identifying cows at risk of early embryonic death as early as 18 days following insemination. The example data show that this new technology, combined with good management approaches, can provide significant value for dairy farms.
Keywords: smart farming, selective dry cow therapy, clinical mastitis, subclinical mastitis, somatic cell count
Abstract. Advancing computer vision (CV) applications in livestock is key to accelerate the on-farm adoption of precision livestock farming (PLF). Our group is executing a five-year project funded by the National Institute of Food and Agriculture (NIFA) of the USDA to: 1) advance CV applications in PLF, 2) attract top talent from engineering, computer science and animal science to PLF, and 3) create a synergetic network of professionals working to solve pressing issues in PLF. Among the outputs of this project are the delivery of recorded and archived webinars on current topics related to CV, the release of reference and benchmarking datasets to facilitate the development and validation of CV tools in PLF, and the creation of analytical challenges based on the published datasets. In this presentation we explain current and planned activities of our network. Specifically, we will summarize the outcomes of the CV webinars delivered up convening the USPLF meeting, the status on available and planned data recording and annotation and current analytical challenges. We also present opportunities for members of the global PLF community to be involved in those activities.
Keywords: computer vision, animal behavior, data sharing.
Abstract. Computer vision systems (CVS) have gained attention in agriculture as a powerful technology for high throughput phenotyping. However, implementing CVS in commercial farms can be challenging due to the massive amounts of data generated and the limitation of Internet bandwidth and latency when using on-premises or cloud computing. To address this challenge, we propose utilizing an edge computing system, which enables real-time monitoring and large-scale data collection by processing data locally at the edge devices. In this study, we developed a low-cost edge computing system designed primarily to video-image livestock animals in farm conditions. The system is based on 3D cameras (n = 29), known as edge devices, each connected to an edge node, here a single-board computer (Nvidia Jetson Nano). The edge nodes communicate through a local Wi-Fi network to an edge server, which also uses an Nvidia Jetson Nano that monitors and manages the edge nodes. The edge computing framework was programmed using the Bash command language interpreter. Log files are generated daily for monitoring the edge system, ensuring the smooth operation of the entire system. One of the key advantages of this system is that it allows for image recording in real-time (24/7) and can process data on the edge system, store it locally, or transfer it to a cloud system. Additionally, other sensing technologies, such as wearable and weather sensors, can be connected to the edge system, expanding the range of data that can be collected. This breakthrough in using high-throughput digital technologies to collect animal and environmental information has the potential to revolutionize how data is collected and processed on farms.
Keywords: camera system, data collection, edge-computing, image recording
Abstract. Swine nursery mortality is highly impacted by the performance of the piglets through the pre-weaning phase of production. Even though the importance of the pre-weaning phase on the downstream post-weaning performance is acknowledged, no predictive modelling is currently being utilized in the swine industry to predict the downstream nursery performance of individual groups of pigs based on their previous preweaning phase. One obstacle to building such predictive models is that health, management, and production data for the pre-weaning and post-weaning phases are collected with separate record-keeping programs and stored in unconnected databases. Thus, the objective of this study was to build a master table that automatically integrates dispersed data collected from one swine production system. After that, the performance of 5 forecasting models was investigated for predicting nursery mortality using the master table containing data on 3,242 groups of pigs (~ 13 million animals) and 44 variables, which concerned the pre-weaning phase of production and conditions at placement in growing sites. After training and testing each model’s performance through cross-validation, the model with the best overall prediction results was the Support Vector Machine model in terms of Root Mean Squared Error (RMSE=0.406), Mean Absolute Error (MAE=0.284), and Coefficient of Determination (R2=0.731). Subsequently, the forecasting performance of the SVM model was tested on a new dataset containing 72 new groups, simulating ongoing and near realtime forecasting analysis. Despite a decrease in R2 values on the new dataset (R2=0.672), the model demonstrated high accuracy (94.52%) in terms of prediction when the mortality of 72 groups was high (5>%) or low (5<%) values. This study demonstrated the capability of forecasting models to predict the nursery mortality of commercial groups of pigs using pre-weaning information and stocking conditions variables collected post-placement in nursery sites.
Keywords: swine, mortality, data-wrangling, forecasting, machine-learning.
Abstract. Highly pathogenic avian influenza (HPAI) is continuously causing significant economic losses with massive depopulations. Airborne transmission of HPAI was suspected, as initial bird mortalities were reported near air inlets of poultry houses. In addition, infected farms were distant, indicating that the viruses carried by dust particles might help the viruses travel for long distances in the environment. The objective of this study focused on simulating the airborne transmission of HPAI by using computational modeling to assess the risk of airborne and deposited avian influenza (AI) carried by poultry-litter dust particles. The Hybrid Single- Particle Lagrangian Integrated Trajectory (HYSPLIT) modeling was used in this study. Data from 168 infected cases in the mid-western area of U.S. were obtained from the Animal and Plant Health Inspection Service (APHIS) and Watt Poultry. The concentration simulation modeling was performed to estimate the airborne and deposited AI concentration carried by PM2.5 dust particles. Results showed that concentrations of airborne AI, deposited AI, and combined AI transmitted to other farms in a day were lower than the minimal infective dose for poultry. In most of the scenarios, the predicted probability of infection showed that Iowainfected farms and turkey poultry houses had the highest infection probability. The findings may provide an understanding of the risk of airborne HPAI virus carried by dust particles and suggest the factors that influence long-distance airborne transmission.
Keywords: Airborne transmission, dust particles, highly pathogenic avian influenza, HYSPLIT modeling,
poultry
Abstract. Chicken coccidiosis is a disease caused by Eimeria spp. and costs the broiler industry more than 14 billion dollars per year globally. Different chicken Eimeria species vary significantly in pathogenicity and virulence, so the classification of different chicken Eimeria species is of great significance for the epidemiological survey and related prevention and control. A new hybrid model integrating Transformer structure and the residual module in convolutional neural network (CNN), named Residual-Transformer-Fine-Grained (ResTFG), was proposed and evaluated for fine-grained classification of microscopic images of seven chicken Eimeria species. The results showed that ResTFG achieved the best performance with high accuracy and computationally efficient compared with traditional models. Specifically, the parameters, inference speed and overall accuracy of ResTFG are 1.95M, 256 FPS and 96.9%, respectively, which are 10.9 times lighter, 1.5 times faster and 2.7% higher in accuracy than the benchmark model. In addition, the results of ablation experiments showed that CNN or Transformer alone had model accuracies of only 89.8% and 87.0%. This study invented a reliable, computationally efficient, and promising deep learning model for the automatic fine-grain classification of chicken Eimeria species, which could potentially be embedded in microscopic devices at an affordable price for producers to improve the work efficiency of researchers and to be extended to other parasite ova, and applied to other agricultural tasks as a backbone.
Keywords: chicken Eimeria classification, deep learning, convolutional and transformer structure,
complementary effect
Abstract. An experimental study was conducted to evaluate the relationship between detectable respiratory disease episodes using SoundTalks technology and multiple PCR testing of weekly oral fluid samples (every pen) as well as weekly air samples (every zone of 12 pens). Pigs used for this study were sourced from a PRRS virus negative and Mycoplasma hyopneumoniae negative breeding herd. All pigs were vaccinated for PCV2 and Mycoplasma hyopneumoniae at weaning. In every pen, three randomly selected seeder pigs were challenged seven days apart with Mycoplasma hyopneumoniae (MHP) and PRRS virus (PRRSv). Respiratory health scores were highly correlated with the air sample and oral fluid respiratory disease PCR test results. Using a stochastic model, air samples were shown to detect MHP at least one week earlier than oral fluids at all oral fluid sample sizes modeled.
Keywords: respiratory disease, cough, oral fluids, air sampling, stochastic modeling
Abstract. This research presents a proof-of-concept for an intelligent biosecurity system specifically designed for use in livestock and poultry production settings. The system, known as the Danish Entry, employs a combination of multiple sensors and an image recognition system to enhance biosecurity measures on the farm. The camera integrated into the system is connected to a computer that runs a visual recognition model capable of identifying potential breaches in biosecurity protocols. The proposed design aims to reduce the likelihood of farm personnel misusing biosecurity procedures and transporting pathogens into the farm. Furthermore, it facilitates slower human traffic flow through the physical barriers within a Danish Entry, thus increasing visual detection accuracy. This system presents a promising solution for improving biosecurity in the livestock and poultry industry using technology and automated approaches.
Keywords: disease, swine, disinfection, pathogen, machine vision
Abstract. Precision Dairy Farming technology for dairy has mostly focused on tools to improve cow care, but new tools are available to improve the care of pre-wean calves and heifers. These technologies apply real-time monitoring to measure individual animal data and detect deviations from normal. On-farm validation of new technologies remains important for the successful deployment of new technologies within commercial farms to understand how the technology can improve dairy calf welfare, performance, and health. Multiple factors exist that are critical in determining if a technology is valuable to farm management. One assessment is determining if the technology can be validated against a gold standard which for calf temperature monitoring, is rectal temperature. This was done by comparing the rectal temperature of calves less than 3 weeks to the rumen-reticular temperature over a 5-hour period using the Farmfit™ system. The first step in the validation of this research was to look at the correlation between sensor temperature and rectal temperature. Lin’s concordance correlation coefficient was strongest at 0.36 when the ruminal temperature reading was used closest to rectal temperature assessment time using the average rumen-reticular temperature of 18:00 and 19:00. The bias correlation to the 1:1 line was 0.988 with an average difference of 0.001°C showing a strong average connection of the Farmfit™ average temperature to the gold standard calves rectal temperature.
Keywords: automation, temperature, dairy calves, bolus
Abstract. Automated milking systems (AMS) already incorporate a variety of milk monitoring and sensing equipment, but the sensitivity, specificity and positive predictive value of clinical mastitis (CM) detection remain low. A typical symptom of CM is the presence of clot(s) in the milk during pre-milking. The objective of this study is the development and evaluation of a neural network (NN) that can detect these clot(s) on pictures of the filters of the milking system after the pre-milking phase. The data for this study was generated by adding debris and/or clot(s) from used milk filters of AMS to milk and passing this milk through a blue circular milk filter mounted in a PVC tube. A camera was mounted in the PVC pipe to take a photo after each pass of milk. In total 696 pictures were taken with clot(s), and 586 pictures without. These were randomly divided into a 60/20/20 training, validation, and testing datasets, respectively, for the training and validation of the NN. A convolutional NN with residual connections was trained and the hyperparameters were optimized based on the validation dataset using a genetic algorithm. The integrated gradients were calculated to explain the interpretation of the NN. The accuracy, specificity, and sensitivity of the NN on the testing dataset were 100%. The integrated gradients showed that the NN identified the clot(s). Further validation and integration on farm in AMS are necessary, but the proposed method is very promising for the inline detection of CM on AMS farms.
Keywords: deep learning, mastitis, automated milking systems, image recognition
Abstract. Monitoring health and production-related parameters of the individual cow in a real-time, cost-effective manner can reduce the costs of production and veterinary care. We will report on a Light Scattering Sensor (LSS) from SomaDetect Inc. The LSS is a self-contained in-line device consisting of a milk channel between a laser and a scattering detector that is attached to the milking line in a dairy parlor or on a milking robot. It requires no operational actions from farm personnel or any consumable reagents. During milking, the LSS collects and transmits a series of images to the cloud. The images are analyzed using proprietary machine learning and artificial intelligence algorithms that currently measure somatic cell count and determine pregnancy status at 18 Days Since Last Heat (DSLH). Other milk and cow attributes are currently being researched. This data, transmitted to the farm in near real-time, can be used to determine udder health and reproductive status of individual cows and groups of cows.
Keywords: light scattering, image analysis, somatic cell count, in-line testing
Abstract. Variation in forage composition significantly decreases the accuracy of diets delivered to dairy cows. Here, accuracy of the delivered diet refers to deviation from the formulated diet and can be improved by using a renewal reward model (RRM) and genetic algorithm (GA) to optimize sampling and forage composition monitoring practices for farm conditions (St-Pierre and Cobanov, 2007a). Specifically, use of quality-controlcharts to monitor forage composition can identify changes in composition for which adjustment in the formulated diet will result in a better match of the nutrients delivered to cows. The objectives of this study were 1) to estimate the corn silage and alfalfa-grass haylage stable time input parameter for the RRM; and 2) optimize farm-specific sampling practices using the RRM and GA. During harvest and silo packing, we recorded the location of the harvested forage from each field-of-origin within the silos on 8 New York dairy farms. During feed-out, we collected silage samples for NIR composition analysis 3x per week for 16 weeks and recorded the field-of-origin based on the location of the forage in the silo. Silage stable time was estimated based on the field-of-origin feeding time or by using k-means clustering of forage sample composition and ranged from 2 to 9 days. Optimal sampling frequency was greater for large farms than small farms. Shorter stable time times resulted in smaller control limits than reported by St-Pierre and Cobanov (2007a) increasing the sensitivity of the quality-control-charts to identify changes nutritional composition of forage.
Keywords: forage, nutrients variability, clustering, optimize sampling practices
Abstract. In recent years respiratory tract sampling techniques are more frequently used in calves to rationalize antimicrobial use and target respiratory health care towards the pathogens involved. This study’s objective was to compare the effects on animal welfare of the three most commonly used respiratory tract sampling techniques in calves, namely deep nasopharyngeal swabbing (DNS), non-endoscopic bronchoalveolar lavage (nBAL), and transtracheal wash (TTW). The metabolic energy for mental performance, based on heart rate and movement data, and other frequently used heart rate variability parameters were determined as a measure for animal welfare. A crossover study was conducted, including five male Holstein-Friesian calves. Individually housed calves were equipped with a combined heart rate and accelerometer sensor which transmitted data to a top view camera. Five sessions were organized, and calves were randomly assigned to one of the five test groups (control (= no animal handling), fixation, DNS, nBAL, TTW). Heart rate variability parameters (mean heart rate, standard deviation of N-N intervals (SDNN), root mean square of successive differences of N-N intervals (RMSSD), ratio of the low frequency (0.04-0.15 Hz) to the high frequency (0.15– 0.4 Hz) (LF/HF ratio), triangular index, SD1 and SD2) were calculated and analyzed using mixed models with calf as random factor. All sampling techniques (DNS, nBAL, TTW) and fixation resulted in an increased mean heart rate compared to the control event. SDNN and SD2 were significantly higher in nBAL and TTW compared to the control. Triangular index showed a discrepancy between TTW, DNS and control. In conclusion, using non-invasively measured heart rate variability parameters showed inconsistent changes. No uniform conclusion could be made based on heart rate variability parameters frequently used in humans for stress monitoring in calves. For the mental component algorithm, it seems that the TTW induced the largest variation in the HR mental component dynamics compared individually per calf with the fixation and control moments. DNS and nBAL seem to induce a smaller and similar variation.
Keywords: heart rate variability, stress monitoring, respiratory sampling techniques, PLF
Abstract. Precision nutrition is an essential part of precision livestock farming, as both pursue enhancing farm profitability, efficiency and sustainability through monitoring animal bioresponses. Precision nutrition in monogastric animals needs tools capable of identifying nutrient imbalances individually over time. Moreover, knowledge of how more efficient ingredients can be synthesized and how feed additives can be intelligently released in the target site within the gastrointestinal tract are also necessary. Our objective was to assess potential tools, combining traditional nutrition with biotechnological, metabolic phenotyping, computational and protein engineering knowledge in a multidisciplinary approach. We evaluated precision nutrition tools based on: (i) metabolic phenotyping and the use of rapid individual biomarkers of amino acid imbalances, (ii) design and modelling of de novo proteins that are fully digestible and meet exactly animal requirements and (iii) smart presentation and release of feed additives in the gastrointestinal tract. Each application was illustrated by a case study. Precision nutrition tools addressed in this work are designed to measure and manage dynamic responses and to equal the dietary nutrient supply to the changing nutrient requirements of animals. Therefore, they can be useful in reducing inputs and outputs for efficient and respectful animal nutrition and health. These tools are necessary prerequisites that must be implemented in future automated and tailored feeding technologies.
Keywords: precision nutrition, monogastric, feed efficiency, biotechnology.
Abstract. To quantify the financial value for variable time horizons a standardized economic index (SEI) was developed, including a cost-to-operate (CTO) with a weighted depreciation across a 12.8-year time horizon to demonstrate long-term value consistency at monthly intervals. Major diet ingredient costs, animal market prices (including slaughter plant value matrix), and monthly inflation data for adjustments to some diet components and preparation/delivery fees were included. Where analytic time frames include projected future periods; available futures contract prices for feedstuffs, pork prices and projected monthly inflation data are used. Technology economic effect differences are calculated using a SEI based on a partial budget model. The SEI is a function of animal performance measures, historical feed ingredient costs, animal market prices and the technology CTO. Animal performance measures utilized in the SEI are average daily gain (ADG), feed conversion rate (FCR), average daily feed (ADF), mortality, and individual animal treatments. Historical monthly market prices and feed ingredient costs are obtained for the most recent 12.8-year time period. In addition to hardware installation costs, a weighted hardware lifespan is determined to calculate the depreciation cost for the CTO value. Cost and price data obtained from various global markets is standardized to a base currency using historical exchange rates of relevance to the currency to the analysis recipient, e.g., dollars, euros, RMB or other currencies. To account for the time-value of money and enable more direct comparison of aggregate time-series data, monthly cost data is adjusted for the corresponding monthly inflation rate.
Keywords: respiratory health, mycoplasma, PRRS, cough, economic modeling, benefit-cost ratio
Abstract. Wastewater generated during animal production contains contaminants that can degrade soil and groundwater quality as well as impact human health. Typically, wastewater is stored in earthen-holding ponds until it can be properly disposed. Subsurface leakage from these ponds is detected using monitoring wells. Monitoring wells have many difficulties inherent with them for effectively detecting leakage. An alternative was developed using resistivity arrays (RA). This technology improves monitoring by measuring multiple sides of a pond using one array and creates a near, real-time flow of information allowing for early leak detection. To automate the technology, a near-surface data analysis protocol was developed that had user-set thresholds to allow scaling of instrument sensitivity. The protocol used a statistical-based process for calibrating the reference data for a wide range of geological and geographical settings. This protocol allowed for the RA technology to continuously measure for leakage and when thresholds are exceeded, send an alert message to managers of potential problems. These advantages provide substantial improvements to production livestock managers for protecting soil and groundwater quality from wastewater pond leakage. The objectives of this paper are to detail the technology development, discuss the advantages with its real-time flow of more responsive information, and illustrate the application of the technology for incorporation with precision livestock management.
Keywords: groundwater quality, resistivity array, wastewater leak detection
Abstract. The objective of this study was to develop an equation-based model for the automation of swine backfat depth (BF), loin eye depth (LED), and intramuscular fat percentage (IMF) estimates obtained from ultrasound images. Commercial pigs (n = 190; Duroc × Large White) were reared in a mechanically ventilated facility in eastern North Carolina. An ExaPad ultrasound machine was used to capture longitudinal images (n = 1168) of the tenth rib at 194 (± 5) days of age. To establish standard measurements for model validation, trained personnel obtained BF and LED measurements manually from the images following standard company procedures. Soxhlet extraction was used to obtain IMF at slaughter. Average BF, LED, and IMF were 14.6 (± 2.6) mm, 63.7 (± 5.5) mm, and 2.0 (± 0.5) percent, respectively. During image processing, the image gradient was used for edge detection to segment ultrasound images into backfat, loin, and rib regions. Segmented images were then used to estimate BF, LED, and IMF. After image quality control and filtering, 1,018 images (190 pigs) were analyzed for trait prediction and linear models were developed. Correlation between measured and predicted values of BF, LED, and IMF were 0.77, 0.76 and 0.51, respectively. Root mean square error of BF, LED, and IMF prediction were 1.65 mm, 3.58 mm, and 0.40%, respectively. Results demonstrate the feasibility of using ultrasound image gradient and an equation-based approach to automate swine backfat depth, loin depth, and intramuscular fat measurements.
Keywords: genetic selection, meat quality, pig
Abstract. The selection of animals to be marketed is largely completed by visual assessment of the animals. The ability to monitor the weight of farm animals in real time would provide important information for better and more profitable livestock farming. In addition, continuous and automatic monitoring of individual animal weight could help identify health and well-being issues. The objective of this study is to develop and evaluate a method based on 3D Convolutional Neural Network to predict weight from point clouds. Intel Real Sense D435 camera placed at 2.7 m height was used to capture the videos of a finishing pig between 20-120 kg freely walking in a holding pen. Point clouds were manually extracted from the recorded 3D video. 3D deep learning architecture is implemented using PointNet method that directly takes 3D points of animal body as input to extract the features and regress those features to estimate the weight of the animal. A total of 1186 images from a total of 249 pigs were used for model training and validating with a 9:1 split. Pearson’s correlation coefficient (r=0.97) was achieved on randomly selected 112 test points clouds. The validation RMSE of the model was 6.79 kg with a test RMSE of 6.88 kg. The results clearly showed that 3D deep learning on point sets has a good potential for accurate weight prediction even with a limited training dataset. Therefore, this study confirms the usability of 3D deep learning on point sets for farm animals’ weight prediction, while a larger data set needs to be used to ensure the most accurate predictions.
Keywords: 3D deep learning, PointNet, weight estimation
Abstract. The estimation of live body weight (BW) of late finishing pigs from a ridge regression model using biometrics collected from an extrapolated 3-dimensional pig model is evaluated in this study. This work compares the predicted BW of 64 pigs with the ground truth BWs manually determined from a scale. Data consisted of partial point clouds acquired from the side view of the pigs using a mobile camera system. The objective of this study was to evaluate the accuracy of finishing pig BW estimation using extracted biometrics (i.e., girth, length, width, height, and flank) from a pig surface extrapolated from a partial point cloud. These biometrics were used as independent variables in a machine learning formula for predicting BW. The root mean squared error (RMSE) was found to be 5.31 kg for the 64 BWs estimated using the extrapolated technique, with an R2 of 0.79. The extrapolation technique was compared to the manual technique by creating a subset of both datasets, which consisted of fifty pigs. Two BW estimation models were created using the subsetted dataset, using the manually collected and extrapolated biometrics. The two BW estimation models found similar RMSE of 4.17 kg and 5.72 kg for the manual and extrapolated datasets. Overall, the process of extrapolating the surface of a pig from a partial point cloud is promising due to the ability to extract any biometrics from only a single partial point cloud, which avoids the disadvantages of manual BW estimation.
Keywords: Blender, camera, machine vision, precision livestock farming, swine
Abstract. The objective was to determine the variance of locomotion score (LS) and growth performance attributable to flooring treatment, hind leg angle, and step length (SL) measured by 3-D image analysis for cattle in slatted feeding facilities. Angus × Simmental steers (N = 189; BW = 352 kg) were blocked by initial BW and assigned to 21 pens. Pens were randomly assigned to 1 of 3 treatments (TRT): concrete slats with no matting, 15 yearold Animat Pebble matting, and new Animat Pebble matting. Individual steers videos were recorded on d 0 using an Intel RealSense depth camera and processed using MATLAB to estimate hind leg angle, SL, and body length (BL). Locomotion scores were assigned using a 0 to 3 scale throughout the finishing phase. The MIVQUE0 option of the MIXED procedure of SAS 9.4 was utilized to estimate the proportion of variance in average LS, overall ADG, and final BW. Variance of average LS was attributed to SL and SL×BL×TRT at 64% and 28%, respectively. For overall ADG, variance was attributable to SL×BL, SL×BL×TRT, and TRT at 38%, 35%, and 25%, respectively. Variables of SL, BL, SL×BL×TRT, and TRT accounted for 38%, 23%, 23%, and 15% of the variance in final BW, respectively. Overall, variance of average LS, overall ADG, and final BW was primarily attributed to SL, BL, TRT, and their interactions. Individual animal differences in structural conformation are related to cattle mobility and growth performance in slatted indoor facilities.
Keywords: 3-D imaging, step length, slatted facilities
Abstract. Estimating performance of beef cattle grazing on extensive rangeland systems is difficult due to labor constraints and stress on animals. Average daily gain (ADG) is an important metric to measure individual animal growth and efficiency, and daily estimates of body weight can help inform management decisions such as supplementation strategy or culling. SmartScaleTM (C-Lock Inc., Rapid City, SD) is a relatively new technology placed in front of existing water tanks that captures front-end weight and RFID tag information while animals are drinking. Though front-end weights greatly increase temporal resolution of individual weight data on animals grazing extensive pastures, no study has quantified the relationship between partial body weight and full body weight across a range of cattle classes. Data collected in 2020-2022 at the Cottonwood Field Station near Philip, SD compared full body weight to partial body weight across mature cows, bred heifers, weaned calves, and yearling steers. Linear regression analysis found a significant relationship (P < 0.001) between partial body weight and full body weight across all classes of cattle (R2 = 0.96), demonstrating the precision of this technology to measure animal body weight in pasture. In addition, season-long SmartScaleTM data was used to present a case study on the application for selecting replacement heifers based on quantile regression. Overall, the ability to measure daily body weights of cattle grazing extensive systems can identify low-performing animals, greatly improve management efficiency, and quantify animal performance.
Keywords: rangelands, precision weighing, beef cattle
Abstract. Respiration rate (RR) is a critical trait associated with animal physiology. It is commonly used to indicate heat stress, respiratory diseases, and welfare for dairy cattle and other animal species. However, this trait is difficult to collect in large-scale operations for both research and commercial settings. This study aimed to develop a computer vision system that accurately predicts the RR of lying Holstein cows using RGB (red, green, blue) and infrared images. Ninety-five videos of thirty lactating cows were collected and had a bounding box annotated over the lying animals’ flank areas (region of interest; ROI). An image-processing pipeline was developed to capture the pixel intensity variation over the ROI and use it to predict the RR. This process utilized Fast Fourier Transform (FFT) to capture the original signal’s frequency domain and select only the frequencies with the five highest power spectral densities. The inverse FFT was then performed on the data, and its peak count was used as the predicted RR. The Root Mean Squared Error of Prediction (RMSEP) and R² were 8.3 breaths/min, 15.8% (RMSEP/Mean), and 0.77, respectively. Applying FFT to the pixel intensity signals from RGB and infrared images was an accurate method to compute the RR of cows in unrestrained conditions.
Keywords: respiration rate, computer vision, spectral analysis, signal processing
Abstract. Climate change is having negative effects on water availability and is a serious challenge to food production around the world. Water scarcity has become a major threat to society, agriculture, and freshwater ecosystems, and the situation is expected to worsen as climate change continues. Sustainability and water efficiency are critical to a poultry industry faced with global production concerns including increased demands for high-quality, affordable animal protein and greater environmental pressures resulting from rising global temperatures, flock heat stress, and limits on water availability. To address these concerns, a commercial sprinkler system used in combination with a cool cell system was evaluated against a cool cellonly system for two summer flocks at Mississippi State University to determine effects of sprinkler technology on cooling water usage, broiler performance, and in-house environments. Environmental and production data were calculated and recorded throughout the flocks. The combination house exhibited a 1.7°C (3°F) (P=0.08) increase in average temperature, numerically lower average humidity (P=0.054), and a 64% (62,039 liters/flock) reduction in average cooling water usage over the cool cell-only house. Litter moisture for the combination house tended to be numerically lower but showed no significant difference at several time points between and across flocks (P=0.11-0.16). Findings are similar to previous reported research and offer additional confirmation that sprinklers in conjunction with cool cells maintain broiler performance while reducing cooling water use, thus lessening the threat to the economic and environmental sustainability of the poultry industry and improving its water efficiency efforts.
Keywords: water efficiency, sustainability, climate change, sprinkler, broiler cooling
Abstract. Respiration rate (RR) is an important physiological parameter highly related to heat stress and respiratory diseases in pigs. Contactless image/video-based RR monitoring has gained widespread interests as it is more animal friendly than traditional ways. However, recent studies mainly focused on monitoring RR for one animal, which is not the practical housing environment for pigs. The main challenge of RR monitoring for group-housed pigs is to detect pigs and select the Region of Interest (ROI) for each pig efficiently. The current study focuses on developing a computer vision-based approach to monitor the RR for group housed pigs towards an automatic way. The proposed method first detects each pig using an oriented bounding box that can capture the boundary between the pig and floor precisely without including many irrelevant pixels. Then the RR was extracted by analyzing the time-varying features extracted from the ROI. The method was validated on the video of four group-housed pigs wearing an ECG (electrocardiogram) belt to collect the gold standard (GS) RR measures. Forty video clips including both day and night occasions were selected to test the method. The comparison between RR obtained by the developed method and the gold standard showed good agreement: MAE, RMSE and correlation coefficient values of 2.38, 3.46 and 0.92, respectively, from four pigs with belts. Future work can further verify the method on the pig without wearing the belt to exclude the effect of the belt.
Keywords: physiological monitoring; oriented object detection; RGB video; animal welfare
Abstract. Ventilation is very important among the many factors that affect the environment in the house. Most pig barns still use traditional ventilation with supplementary cooling systems, but this ventilation model cannot guarantee that each pig in the house receives appropriate fresh air. Therefore, this study evaluated an alternative supplementary cooling system, namely the precision air delivery system. This system is composed of a main duct and a distribution duct, which extracts outdoor air through a fan and then blows air to the pigs through the distribution duct. It can effectively eliminate the excess heat in the house and ensure that each animal is in a comfortable thermal environment. In this study, after proposing an improvement plan after field research, the simulation was verified by computational fluid dynamics techniques to investigate the effects of three study parameters (ventilation rate, air supply duct diameter and air supply angle) on the cooling performance of the precise air supply system by taking a standing pig body as an example and finding the best design solution within the study range by response surface analysis (ventilation rate of 400 m3/h, duct diameter of 0.1m, duct angle of 53°) within the study range was found by response surface analysis, so as to achieve the purpose of improving the production efficiency of pig farming.
Keywords: pig house, ventilation, CFD, precision air supply system, thermal environment
Abstract. Systems to continuously monitor broiler chicken welfare during grow-out are emerging which rely on sensing and machine learning. Audio analytics, one such system, is a promising complement to video analytics and environmental sensing. Machine learned models developed in academic settings often do not perform well in commercial settings, so there is interest in practical examples of audio analytic use cases developed in commercial settings. This paper describes AudioT INCs audio analytic system developed in commercial growout house as part of FFAR’s Smart Broiler initiative. A description of the data acquisition, model development, and model results is shared around a practical example of respiratory disease detection and tracking.
Keywords: real-time recognition, audio analysis, spectral analysis, signal processing, poultry welfare
Abstract. Broilers need to access feed for their daily nutrient. Therefore, feeding behavior is one of broiler chickens’ most critical welfare indicators. Since feeding costs remain the biggest cost in raising broilers, understanding feeding behavior can provide important information regarding the usage of poultry resources and insights into farm management. Monitoring poultry behaviors is typically performed based on visual human observation. In spite of the successful applications of this method, its implementation in large poultry farms is time-consuming, laborious, and expensive. Thus, there is a need for automated approaches which can overcome these challenges. On the other hand, there is no research on the feeding time estimation of individual broilers in commercial farms. Consequently, this study aimed to evaluate the feeding time of individual broilers by a Convolutional Neural Network (CNN)-based algorithm. To achieve the goal of this research, videos from a poultry farm were acquired, and 1500 labeled images were used for training the CNN model. Three 1-minute labeled videos were applied to evaluate the proposed algorithm’s performance. The algorithm achieved an overall feeding time estimation of each broiler per visit to the feeding pan accuracy of 87.3%. In addition, the obtained results prove that the proposed algorithm can be used as a real-time tool in poultry farms.
Keywords: broiler, feeding time, CNN, image processing
Abstract. Broiler mobility is a vital welfare indicator which may influence their ability to access feed and water. Classical broiler mobility assessment methods are laborious and cannot provide continuous insights into individual bird’s conditions. In this paper, we proposed a semi-supervised Deep Learning (DL) model, YOLOv5, combined with our newly developed variant of the conventional Deep Sort tracking algorithm, Neo-Deep Sort, for individual broiler detection and trajectory tracking, respectively. Initially, 1,650 labeled images from five different days were employed to train the YOLOv5 model. Through the semi-supervised learning (SSL) which significantly reduced the lengthy data labelling process, this narrowly trained model was then used for pseudo-labeling 2,160 images, of which 2,153 (99%) were successfully labeled. Thereafter, the YOLOv5 model was fine-tuned on the newly labeled images. Lastly, the meticulously trained YOLOv5 and the Neo-Deep Sort algorithm were applied to detect and track 28 broilers in two pens and consequently categorized them in terms of hourly and daily moving distances and speeds. As a result of the SSL, the YOLOv5 model’s mean Average Precision (mAP) in identifying birds with a 50%-100% confidence increased from 81% to 98%. As compared with the manually measured covered distances of broilers, the combined model provided individual broiler’s hourly moved distances with a validation accuracy of about 80%. Eventually, individual and flock level mobilities were quantified while overcoming the occlusion, false and miss detection issues. The vision-based algorithm developed in this study may serve effectively to track indicators critical for broiler production performance and welfare.
Keywords: broiler welfare, mobility, YOLOv5, semi-supervised learning, Neo-DeepSort
Abstract. Gait scoring is a useful means of evaluating broiler production efficiency, welfare status, bone quality, and physiological traits including leg health status. The research objective was to characterize spatiotemporal and three-dimensional (3D) locomotive behaviors of individual broilers by using deep learning algorithms on RGB and 3D depth top-view images. Ross 708 broilers were placed on a platform specifically designed for gait-scoring and manually categorized into one of three numerical scores. RGB and depth cameras were installed on the ceiling to capture top-view videos and images. Four birds from each of the three gait score categories were randomly selected out of 70 total birds scored for video analysis. Bird moving trajectories and 16 locomotive behavior metrics were extracted and analyzed via the developed deep learning models. The trained model gained 100% accuracy and 3.62±2.71 mm root mean square error on estimating a key point on the back of each broiler, indicating precise recognition performance. Broilers with lower gait scores (less difficulty walking) exhibited more obvious lateral body oscillation patterns and moved significantly or numerically faster and covered more distance in each movement event than those with higher gait scores. In conclusion, the approach presented, which differentiates between selected spatiotemporal and 3D locomotor behaviors, showed acceptable performance for tracking broilers and can be a useful research tool for automating individual broiler gait scoring. Further development with more images is recommended.
Keywords: behavior recognition, poultry, animal welfare, artificial intelligence
Abstract. The present study aimed to develop predicting algorithms to identify the effectiveness of environmental enrichment (music and light stimuli) in reducing leg disorders in broiler chickens. The trial was carried out in one flock of broiler chickens on a poultry farm using four broiler houses. A total of 1,600 broiler chickens were used in the trial. Twenty broiler chickens (ten males and ten females) were caught and weighed, and footpad dermatitis, hock burn, valgus-varus deformity, and crooked toes were observed. The gait score was assessed once a week for five weeks. Data mining was applied using the decision tree classification algorithm based on the Iterative Dichotomiser 3 algorithm (J48 in the Weka® environment) and the cross-validation operator. Broiler weight was the decisive factor in predicting leg disorders. Environmental enrichment was the most critical factor in footpad dermatitis (81% accuracy). The vital factor for predicting valgus/varus is age. The environmental enrichment was the most crucial factor determining crooked toes’ incidence (70% accuracy). The critical aspects affecting gait score were broiler weight and sex (decision tree with 82% accuracy), and the incidence of hock burn was related to weight, environment enrichment, and sex (77% accuracy). The algorithms predicted the leg disorder and lameness in broilers subjected to the stimuli utilizing environmental enrichment.
Keywords: real-time recognition, cough analysis, spectral analysis, signal processing
Abstract. Cattle identification plays an irreplaceable role in understanding disease trajectories, animal traceability, and animal ownership assignment. Although traditional non-biometric methods of cattle identification have been used extensively, issues with retention, cost and intentional removal remain. Here we developed a robust Deep Learning based cattle facial biometric feature identification system using cattle head images extracted from video as the input. The developed methodology differentiated the cattle face from the background via a combination of instance and semantic segmentation. The images as video frames were then normalized to ensure consistency in the sense of image size and cattle head location. The output of the system was a set of identified biometric features for each image. A case study was conducted using videos of 25 cattle from 2-year-old beef cattle recorded at the University of Sydney John Bruce Pye Farm. The videos were pre-processed, and a database of 5,000 high quality frontal images was created. The performance of different neural networks previously used for human facial feature identification was then compared. The MobileNetV2 neural network demonstrated the best performance for automated identification of cattle facial key regions achieving 99% precision and recall for muzzle detection, and 98% precision and recall for eye detection. Thus, the proposed approach enables an automated computation of a range of biometric features including, and not limited to, the relative distances between eyes, between eye and muzzle and the angle between different facial features. The availability of automatically calculated biometric features enables work towards the identification of individual cattle.
Keywords: biometric identification, object detection, cattle traceability, image segmentation, deep learning
Abstract. Computer vision systems have been proposed as an automated and non-invasive alternative to identifying animals with distinct coat color patterns, but there are limited applications in solid-colored herds. The aim of this study was to identify dairy cows using infrared image to identify keypoints located at specific anatomical landmarks (e.g., bony prominences). Two-thousand ninety-seven images (top-down view) were collected from 25 dairy cows. Seven keypoints per cow were manually added on the dorsal area. These locations were bony prominences [ hip (left and right), pin bone (left and right), tail head, sacral and cervical vertebrae] that can remain constant when cows change body condition score. We used the Euclidean distance between keypoints to generate biometric features for each cow and analyzed the data using a multi-layer feedforward artificial neural network (ANN). The ANN model’s hyperparameters were defined using a grid search method, which included 6 activation functions, 76 hidden layers, 5 input dropout ratios, 5 levels of lasso regularization (L1), and 5 levels of ridge regularization (L2). The model was built using a 5-fold cross-validation approach, with 1,690 images selected for training and 407 images used for testing. The final model achieved an accuracy of 80%, precision of 71%, recall of 74%, and an F1 score of 80%. These results suggest that keypoints located on the dorsal body surface can be an effective alternative for identifying individual animals that lack distinct coat color patterns.
Keywords: animal identification, deep learning, euclidean distance, body biometrics
Abstract. Individual animal identification is extremely important in dairy production. Accurate animal identification is not only important for farm management, but also for issues of food security and consumer trust. Computer vision has been proposed as a reliable, non-invasive system to recognize animals, but there is limited research on their capacity to detect the same individual at different stages of life. The potential for computer vision systems (CVS) to recognize an adult cow from her images as calf will allow for comprehensive animal tracking and reliable traceability systems. The objective of this study was to develop a CVS that utilizes images collected from Holstein calves in the first few weeks of life to identify the same individuals after a year of growth. To train the model, top-down view infrared images of 10 calves (1 to 4 wk of age) were collected on four separate days and segmented for the calf body, resulting in 200 images per calf. The images were used to train a deep neural network (Xception) for individual identification. The trained model was tested on 20 infrared images of each animal collected from top-down view after one year (60 wk of age). All analyses were implemented in Python using the open-source framework Tensorflow and Keras. The precision and recall of prediction for identifying individual calves were 0.78 and 0.76, respectively. These results demonstrate the potential of CVS for animal identification, even when images used for training are collected much earlier on the animals’ life and during their growing phase.
Keywords: computer vision, dairy calves, identification
Abstract. Supplementation of beef cattle can be used to meet both nutrient requirements and production goals; however, supplementation costs influence farm profitability. Common supplementation delivery strategies are generally designed to provide nutrients to the mean of the group instead of an individual. Precision individual supplementation technologies, such as the Super SmartFeed (SSF, C-Lock Inc., Rapid City, SD), are available but are generally cost prohibitive to producers. These systems require adaptation or training periods for cattle to utilize this technology. The objective of this research was to assess training and adoption rates of three different groups of cattle (suckling calves, weaned steers, replacement heifers) to the SSF. Successful adaptation was determined if an individual’s supplement intake was above the group average of total allotted feed consumed throughout the training period. Suckling calves (n = 31) underwent a 12-d training period on pasture; 45% of suckling calves adapted to the SSF and average daily intake differed (P <0.0001) by day of training. Weaned steers (n = 79) were trained in drylot for 13 d. Of the weaned steers, 62% trained to the SSF, and average daily intake differed (P < 0.0001) by day of training. Replacement heifers (n = 63) grazed tall fescue pastures and had access to SSF for 22 d of training. The success rate of replacement heifers was 73%. For replacement heifers, daily intake did not differ (P < 0.0001) by day of training. Results indicate production stage and training method may influence young cattle adaptation to precision technologies.
Keywords: precision supplementation, technology adaptation, beef cattle
Abstract. Comprehensive livestock tracking and behavioral characterization in extensive systems is technically challenging and expensive. Some technologies and data strategies based around proximity information may be more affordable. This paper brings together experiences from two major PLF projects involving cattle in extensive U.S. rangelands and sheep in extensive UK mountains and considers proximity technology for two resources, water in dry rangelands, and supplementary feed in pregnancy, respectively. Opportunities to characterize useful livestock variables include presence/absence, diurnal patterns, use of resources and changing use patterns. Results covering supplementary feed, used fixed Bluetooth Low Energy (BLE) readers arrayed around feeding points, 48 Blackface and 50 Lleyn ewes on 33ha of grazing that wore small (c14 g) BLE beacons. Beacons on ewes communicated identity and RSSI (Received Signal Strength Indicator) via receiving readers, pushing data in near-real time via LPWAN to an ArCGIS Online database. Differences in proximity at feeding areas were found for breed and age and patterns of activity over 24-hour periods, supporting the view that BLE technology covering only proportions of grazing areas could be useful for management purposes. For water access in arid rangelands, 11 cows in a 480ha paddock wore NoFence virtual fencing collars with GNSS real-time tracking using cellphone communications. Daily patterns of proximity to the only water source derived from GNSS data support the view that useful information could be provided by BLE proximity systems at lower cost than GNSS collars. Proximity approaches alone provides less information than GNSS systems.
Keywords: proximity, Bluetooth, GNSS, extensive systems, cost/benefit
Abstract. Bovine Respiratory Disease (BRD) is a multi-pathogen syndrome that manifests as a result of stress caused by handling, transport, or an underlying viral infection. At an incidence rate of 18-21%, BRD is the costliest disease in beef and dairy cattle in North America, leading to almost a billion dollars in annual losses. Due to the complexity of the disease, management and therapy are challenging. Despite efforts to advance BRD diagnostics, selected treatment protocols (antibiotics) continue to have a high failure rate. This issue is further complicated by the increasing resistance of BRD pathogens to standard antimicrobial agents. We have developed a pen-side diagnostic assay that detects three different types of bacteria (Pasteurella multocida, Mannheimia haemolytica, and Histophilus somni) from nasal swabs of cattle. Our assay can be conducted on the farm and provide results in the form of a color change that is visible to the naked eye within an hour. The assay demonstrates 60-100% concordance when compared to lab-based analysis. These assays use loop-mediated isothermal amplification (LAMP) to detect DNA from the sample and thus, can be easily reconfigured for the detection of viruses or antimicrobial resistance genes. They can also be adapted to other diseases such as mastitis or other animals such as pigs or poultry. Widespread use of such pen-side diagnostics can help management decisions for individual animals, reduce the spread of antimicrobial resistance, and increase animal welfare.
Keywords: diagnostics, bovine respiratory disease, loop-mediated isothermal amplification, pen-side
Abstract. Australian beef cattle are located across a diverse landscape, and our new, increasingly extreme climate is impacting the sustainability of this typically extensive production system. Resilient cattle and complementary management approaches are required for this new environment. Previous work has used milk yield variability as an indicator of dairy cattle resilience linking low variability in production with greater resilience; however, there is a paucity of work using the same method for beef cattle. Here we determine growth variability within a herd using 37,621 live weight observations from 3,813 cattle over two years. Liveweight (LW) was measured using the Optiweigh system, where LW was obtained opportunistically inpaddock as cattle access a molasses-based lick block. Firstly, data were pre-processed in three steps, and growth curves for each animal were created using a linear mixed model. Each animal’s residual standard deviation (SD) of LW (SD of the residuals about the fitted model) was calculated as a measure of resilience. The lowest, mean, and highest residual SD were 4.41 kg, 10.24 kg, and 21.67 kg, respectively. Cattle with the lowest residual SDs were less variable around their LW growth trajectory, indicating they could cope with extreme climatic events. In conclusion, the residual SD is a promising resilience indicator, with the reasons for the diversity in this indicator forming the basis for further work.
Keywords: Climate extremes, live weight profiles, resilience, beef cattle
Abstract. Measuring grazing beef cattle dry matter intake (DMI) is a significant challenge. The DMI value is required to determine stocking rates and supplementation requirements. The variation in estimated DMI of grazing cattle introduces tremendous error at the herd level since estimated DMI is based on full body weight (BW) multiplied by a percentage of BW. The BW percentage varies depending on animal class, production phase, and forage nutrient composition. Although Animal Unit Months are used to simplify this calculation fo rangeland systems to determine the duration that cattle can graze, there is tremendous opportunity to leverage precision technology to account for the individual animal variation and subsequent impacts on herdlevel decisions. Therefore, the objective of this study was to build a precision system model (PSM) to evaluate the differences in modeled DMI compared to the traditional herd-level method using initial body weight (BW). The model evaluated hectares needed and total forage consumption using daily individual heifer BW (kg) (n = 60; precision data) grazing dormant native rangeland for 175 days in South Dakota using a SmartScaleTM. The traditional versus the PSM method resulted in an additional 73.41 ha required. Sensitivity analysis of percent BW (1.8 to 2.7%) resulted in a 33 and 39% difference in forage consumption and hectares required, respectively. Applying precision data provides more precise DMI estimates and shows the advantages and disadvantages of herd-level estimates. The use of PSM helps to identify high-leverage precision tools to minimize a performance gap like overgrazing extensive rangeland systems.
Keywords: grazing, dry matter intake, modeling, beef, rangelands
Abstract. Automated animal activity recognition (AAR) has succeeded dramatically due to recent sensing technologies and deep learning advances, enhancing animal health and welfare. Since animals need to be monitored over a long period, factors influencing the energy consumption of sensing devices must be considered carefully. As a critical factor, the sampling rate greatly affects energy usage, battery life, and data storage. To reduce energy costs, existing works often lower sampling rates. However, when the sampling rate falls below a limited threshold, the recognition performance would degrade rapidly due to missing many relevant signals. Therefore, this study proposed a novel correlation distillation-based information recovery (CDIR) method to improve the performance of AAR at low sampling rates. Specifically, we took two convolutional neural networks trained using data having higher and lower sampling rates as the teacher and student models, respectively. The CDIR enabled the student to mimic correlations within teacher features, facilitating missing information recovery. To evaluate its effectiveness, we conducted experiments on a public dataset acquired from six horses using tri-axial accelerometers and gyroscopes with 100 Hz. Data having low sampling rates were obtained by down-sampling the original data at different frequencies (e.g., 50, and 25 Hz). The experimental results demonstrated that our CDIR remarkably boosted the model trained on low sampling rate data (e.g., percentage-point increments in the precision, recall, F1-score, and accuracy of 2.98%, 3.22%, 3.05%, and 1.89%, respectively, for the 12.5-Hz data) while outperforming the existing KD algorithms. This inspired the development of energy-efficient animal monitoring systems.
Keywords: behavioral classification, deep learning, resampling, knowledge distillation
Abstract. Social dominance in cattle is important when resources are scarce and competitive situations occur such as in a queue in front of an automated milking system (AMS). We aimed to 1) create and validate an algorithm to automatically register waiting time in front of an AMS (WT_AMS) for individual cows and 2) study the effect of dominance on observed WT_AMS. Our research took place on a commercial dairy farm in the Netherlands housing 110 Holstein Friesian dairy cows and operating a two stand GEA MIone AMS. Cows were fitted with a NEDAP SmartTag Neck that included cow location. Fifteen one-hour-long observation periods took place during which three researchers noted the time an animal came into a preselected open waiting area in front of the AMS and the time of either leaving the waiting area or entering the AMS. During the time an animal was in the waiting area dominance behavior performed or received by the focal animal was registered. An algorithm was developed to determine the WT_AMS based on location data. WT_AMS for observations and algorithm were strongly correlated (Spearman’s rank-order correlation: r=0.828; p=0.000; n=112). A weak negative correlation was found between dominance and waiting time in front of the AMS (r=- 0.248; p=0.01; n=66). In conclusion, the algorithm can be used to automatically assess WT_AMS accurately, and dominance behavior was found to have a small effect on waiting time in front of the AMS. More research is needed to determine the effect, for instance, of disease on individual WT_AMS.
Keywords: dairy cow, behavior, dominance, waiting time, location system
Abstract. Modern hog production has no current means to collect swine pulse or respiration rate other than to train and task an individual to conduct manual data collection. Respiration and pulse rates are key physiological indicators that are correlated with multiple health issues, including heat stress. However, they require significant manpower to measure and record, and these data are impractical to collect for large commercial operations. Based upon their previous livestock instrumentation experiences, researchers at Purdue University have developed a vaginal device to collect deep body thermal data, which is believed can also be used to determine pulse and respiration through the inclusion of acoustical measurement. A fresh prototype design will add a microphone and audio processor to the current intravaginal thermal device. Heart and diaphragm noises within the body will initially be compared to externally derived signals and human measurement, to baseline the premise’s performance. The new device, used within a farrowing barn environment, could automatically transmit these new sow data channels to a central computer at regular intervals, so that real time decision making could occur. This paper will detail the background and design of the current device, the developmental tests that have been performed so far, and the design focus for a next generation prototype device.
Keywords: body temperature, intravaginal sensor, precision livestock instrumentation, pulse rate, respiration rate
Abstract. This work explores possibilities for monitoring animal welfare in an objective way by measuring physiological variables. Worldwide pneumonia is very common among calves. Sampling techniques have been developed to detect such pneumonia. The concern is to know whether these techniques impact animal welfare. The objective of this paper is to describe how we detect the effect of these sampling techniques of the respiratory tract on calf welfare. First experiments were conducted on three male Holstein-Friesian calves under thermal controlled experimental conditions. Following stressors were applied: deep nasopharyngeal swabbing (DNS), non-endoscopic bronchoalveolar lavage (nBAL), transtracheal wash (TTW), blood sampling and animal fixation. Each calf was wearing a sensor measuring heart rate (BPM, 3 Hz) and activity (x-, y-, zaccelerations; 26 Hz). A data-based mechanistic model adapts to each individual calve and next, the real-time model adapts to individual variations during possible stressful sampling techniques. The model decomposes the measured total heart rate into different components, namely heart rate components required for: the basal metabolism, the physical activity, and finally the mental component. The data-based mechanistic model for the dynamic response of the mental component during a sampling technique exhibits an R2 = (95 ± 4) % and Young Identification Criterion YIC = (-7.4 ± 3.4). The individual model parameters for each calf vary from b0 = 0.10 ± 0.03 bpm-1 to b0 = 11.9 ± 0.6 bpm-1, confirming individually different responses of each calf as expected.
Keywords: physiological data, stress monitoring, respiratory sampling techniques, PLF
Abstract. The personality traits in cattle have been traditionally measured in highly controlled experimental settings. Precision technologies can measure a myriad of variables continuously, including feeding behavior, activity, and production measures. The utilization of precision technologies grants the opportunity to explore behavioral patterns that may be related to animal personality traits in a natural setting. This study’s aim was to investigate the relationship between the behavioral responses of the individual from personality tests and the different measures from precision technologies. In this narrative review, we found that growth, intake, activity, and milk production all have variable relationships with certain personalities and behaviors that are identified from standardized tests. These relationships between personality traits and the behaviors measured by precision technology indicate that stable differences among individuals can be measured and identified in natural settings.
Keywords: temperament, coping styles, ruminants, animal welfare
Abstract. Air inside pig barns is characterized by high concentration of ammonia (NH3) and particulate matter (PM). The high number of pigs reared into large scale farms contributes to the deterioration of air quality inside the barns, posing a health risk to animals and workers. The same airborne pollutants then are emitted outside, causing pollution in the surrounding environment. The LIFE-MEGA project implemented in heavy pig houses a control unit equipped with an artificial intelligence firmware able to continuously monitor the concentration of NH3 and PM inside the barns and activate the operation of two different abatement technologies (dry filter and wet scrubber) for air quality control. Thanks to a reduction of airborne pollutants concentration, positive effects on animal health and welfare were observed.
Keywords: air quality, scrubber, AI microclimatic tool, pig farm
Abstract. Energy consumption of livestock production is one of the main concerns in breeding industry and in global greenhouse gas emissions. This study designed a new dehumidification system that took full advantages of the characteristics of natural low temperature in cold winter with low indoor heat loss. This paper established models to predict the performance of this dehumidification system based on the deep learning method. The results showed that deep learning models had the best prediction under the hyperparametric combination when hidden layers number was 10, neurons number was 200, loss function was MAPE and optimizer was Adam. The predicted values of LSTM and GRU were consistent with the real values, and R², RMSE and MAPE of GRU model were 0.9152, 3.07% and 2.38%, respectively. This method can accurately predict the performance of dehumidification system, which can provide optimal working condition of dehumidification system.
Keywords: Livestock house, cold region, dehumidification system, condensation dehumidification, deep learning algorithm
Abstract. This paper describes a calculation model for the prediction of methane and ammonia emissions from fattening pig houses. This model was validated with continuous and discrete measurements from two manure management systems (MMS) (long storage (LS) in deep pits and short storage (SS) by daily flushing of a shallow pit with sloped walls). The average calculated methane and ammonia emissions corresponded well with the measured values. Based on the calculated and measured results, the average calculated CH4 emission (18.5 and 4.3 kg pig-1 yr-1) corresponded well with the continuous measured values using sensors (15.9 and 5.6 kg pig-1 yr-1) and the discrete measurements using the reference method (22.0 and 3.1 kg pig-1 yr- 1) for LS and SS, respectively. The average calculated NH3 emission (2.6 and 1.4 kg pig-1 yr-1) corresponded well with the continuous measured values using sensors (2.6 and 1.2 kg pig-1 yr-1) and the discrete measurements using the reference method (2.7 kg pig-1 yr-1 and 1.0) for LS ad SS, respectively. Based on the results of the reference measurements, the approximate reduction potential of these measures for CH4 and NH3 emissions is 86% and 63%, respectively. The upgraded model with robust calculation rules, extensive validations and a simplified interface can be a useful tool to assess the current situation and the impact of mitigation measures at the farm level.
Keywords: methane emission, ammonia emission, modelling, manure, pig house
Abstract. This paper describes the development and validation of a housing system for growing-finishing pigs with an integrated solution for source oriented measures to reduce emissions of ammonia, greenhouse gases (methane), and odor. In the development phase a simple calculation tool was used to make an estimate of the ammonia emission that could be expected. In the validation phase the system was tested on two farms in a case control setup. Preliminary studies were done to determine the effect of cooling and flushing on the ammonia emission. From this study the following can be concluded: – a rather simple model can be very useful in design studies to reduce (ammonia) emissions; – cooling of manure is effective in reducing ammonia emissions, but is less suitable in combination with a biogas digester; – ammonia emission only showed a minor rise during flushing of manure; – the designed low emission housing system, with daily removal of manure, small emitting area and diluted manure, lowered ammonia emissions by 54%, methane emissions by 90%, and odor emissions by 28%; – reductions of ammonia and odor can be increased by good management of the whole system.
Keywords: ammonia, methane, odor, emissions, pig housing, indoor climate
Abstract. To improve the indoor environment conditions in pig buildings, an innovative prediction model with three layers using long short-term memory (LSTM) was developed to forecast indoor environment conditions including five factors of the gas concentrations of ammonia (NH3), carbon dioxygen (CO2) and hydrogen sulfide (H2S), and thermal environment conditions of air temperature (PLS T) and relative humidity (PLS RH). In the LSTM model, there are 5 inputs and outputs representing for the five environmental factors in history and the next time step, respectively. The auxiliary influenced factors with high correlations with the outputs were selected to attend the calculation in fully connected layer. 53 days collected data in wintertime and summertime were used for the model training and testing. It was shown that the model with hyperparameters of 400 neurons, 1 time lag, and 0.008 learning rate has optimal performances both in summertime and wintertime. Compared with other two different types of models of ANFIS and MLR, the LSTM model has the best performances of RMSE, MAPE and R2 for the concentrations of NH3 in wintertime, H2S in summertime, PLS T and PLS RH both in summertime and wintertime.
Keywords: thermal environment conditions, gas concentrations, environment control, pig building, long
short-term memory.
Abstract. Indoor arenas are semi-indoor structures located on equine farms and facilities for exercising horses, exhibiting skills during competitive events, and other equine related activities. These spaces do not always include mechanical ventilation or stirring fans and occupancy by horses and humans can be sporadic and inconsistent, which creates a challenging space for understanding and predicting airflow. Typically, indoor arenas have a sand-based footing over which the horse travels. The impact of the hooves can cause dust to become a concern within the facilities. Spatial variability analysis in previous research indicates the variability of air speeds in these facilities is not impacted by design characteristics of the indoor arena. In addition, air speeds were low enough to be considered still air conditions. To further understand the interior environment within indoor arenas, monitoring was conducted at 15 facilities within 200 kilometers of Lexington, KY. Environmental monitoring of air speeds took place over 7 days in the winter and summer to examine temporal variability. Air speed data was collected every 5 minutes using the HOBO RX3000 Remote Monitoring Station with the HOBOnet Ultrasonic Wind Speed and Direction Sensor. Similar results to the spatial variability indoor arena characterizations were observed during the environmental monitoring with air speeds being below the threshold for still air in livestock facilities (0.51 m/s). Sensor technology and implementation provides a better understanding of airflow and how indoor arena design can impact and improve it.
Keywords: equine, indoor arenas, spatial variability, temporal variability
Abstract. An evolution of ventilation options is underway by the egg industry to keep pace with the advancing transition to cage-free production. The presented study analysed performance of four ventilation schemes within a commercial cage-free hen house using the computational fluid dynamics (CFD) modelling approach. The analysis aimed to refine ventilation design of cage-free hen housing to assure bird welfare and improve the capacity to constrain airborne disease spread. In total, four three-dimensional CFD models of one-eighth portion of a floor-raised hen house were developed in full-scale to compare the performance of a standard ventilation configuration with three alternative designs. To assess challenging indoor environmental conditions within the four ventilation schemes, the performance of ventilation during freezing winter weather was investigated. In addition, a realistic number of birds were modelled individually with simplified geometrical shape with thermal physical settings to account for the sensible heat generated by animals. The dispersal behaviour of surrogate virus particles was analysed coupled with airflow patterns at critical locations. One of the highlights in this study was the emphasis on assessing the environmental conditions at the bird level, in terms of efficient ventilation, airflow, temperature, and disease spread, which provided insights for precision environment management of livestock and poultry facilities.
Keywords: cage-free hen housing, ventilation options, computational fluid dynamics model, precision
environment management, airborne disease spread
Abstract. Real-time and automatic detection of chickens (e.g., laying hens and broilers) is the cornerstone of precision poultry farming based on image recognition. However, such identification becomes more challenging under cage-free situation compared to caged hens. In this study, we developed a deep learning model (YOLOv5xhens) based on YOLOv5, an advanced convolutional neural network (CNN), to monitor hens’ behaviors in cage-free facilities. More than 1000 images were used to train the model and additional 200 images were adopted to test it. The one-way ANOVA and Tukey HSD analysis were conducted using JMP software (JMP Pro 16 for Mac, SAS Institute, Cary, North Caronia) to determine whether there are significant differences between the predicted number of hens and the actual number of hens under various situations (i.e., age, light intensity, and observational angles). The difference was considered significant at p<0.05. Our results show that the evaluation metrics (Precision, Recall, F1 and mAP@0.5) of the YOLOv5x-hens model were 0.96, 0.96, 0.96 and 0.95, respectively, in detecting hens on the litter floor. The newly developed YOLOv5x-hens was tested with stable performances in detecting birds under different lighting intensities, angles, and ages over 8 weeks. For instance, the model was tested with 95% accuracy after birds were 8 weeks old. However, younger chicks such as one-week old birds were hard to be tracked (e.g., only 25% accuracy) due to interferences of equipment such as feeders, drink lines, and perches. According to further data analysis, the model performed efficiently in real-time detection with an overall accuracy more than 95%, which is the key step for individual birds’ tracking for evaluation of production and welfare. However, there are some limitations for the current version of the model. Error detections came from highly overlapped stock, uneven light intensity, and images occluded by equipment (i.e., drinking line and feeder). Future research is needed to address those issues for a better detection. The current study established a novel CNN deep learning model in research cage-free facilities for hens’ detection, which provides technical basis for developing a machine vision system for tracking individual birds for evaluation of animals’ behaviors and welfare status in commercial cage-free houses.
Keywords: egg production, cage-free system, precision farming, deep learning algorithms
Abstract. In order to accurately and efficiently analyze spatial utilization and spatial distribution of chickens, and realize the automatic monitoring of vertical space motion behavior in large cage aviary unit system, a spatial behavior monitoring system based on UHF RFID (Ultra High Frequency Radio Frequency Identification) was developed and tested. The monitoring system was mainly composed of passive electronic tag, antenna, UHF reader, industrial computer and bundled software. The optimization of this monitoring system was achieved by combining RFI distance equation and filtering processing method. The monitoring system was used to continuously record 16 chickens for 5 days in large cage aviary unit system, and cameras were recording for manually labeling as a reference. The results showed that: 1) The uniform distribution of RF energy in each layer of the test unit could be ensured when the antenna transmission power was 18.5 dBm. 2) The average accuracy rate of this monitoring system was 87% ± 3%, which basically met the requirements of field test. 3) The used median filtering method with sliding window size n = 5 could significantly improve the accuracy and stability of the system and reduced the lag effect of filtering on the motion change of vertical space. The system accuracy after filtering was 95%. This study will be helpful for monitoring of the vertical space motion behavior of chickens, and analyzing the spatial distribution and facility utilization, which provided scientific basis for improving the production performance and welfare level of chickens in large cage aviary unit system.
Keywords: multi-tier rearing system, three-dimensional space motion, UHF RFID, median filter
Abstract. Activity is considered one of the most promising animal-based measures (ABM) of animal welfare and health. However, tracking activity is a challenge in the barn. To date, different techniques have been considered. Video technology can detect pixel-based activity via various algorithms, but is costly to install. Passive infrared sensors (PID) could provide a simple and practical alternative supporting the farmer. To validate these techniques, 9 pens of pullets were observed 24/7 for 33 days. The sensors were placed so that they each focused on a pen of 56 animals in 9.2 m² area. Pens differed in genotype and treatment (flicker frequency of the LED light). Data were measured continuously at 1-min intervals. Values were tested in empty pens and recorded afterwards in occupied pens, and the readings represented the entire range of 100% output during animal observation. The PID sensors showed a mean activity during the light phase of 21.74% and during the dark phase of 4.73%. Measurements from the PID sensors, video-based analyses, and ethological scan sampling observations have been analyzed. The correlation of PID and ethological data ranges between r = 0.512 to 0.845 (P ≤ 0.001) reflecting a strong correlation throughout the data set. Video based validation reveals lower correlation coefficients within the six focal days based on one pen (r = 0.37 to 0.684, with 3 other days P ≥ 0.3). Further data cleaning should be applied. The measurement of activity in the pen offers simple approaches of the PPF to improve animal welfare, such as the derivation of activity for the enhancement of animal health or in relation to the adequate occupation of the animals with enrichment materials.
Keywords: infrared sensors, activity, chicken, welfare
Abstract. Floor egg-laying behavior (FELB) is one of most concerned problematic behaviors in commercial cage free (CF) housing systems, leading to increased mislaid eggs, which range from 0.1-10% of total daily egg production. In addition, mislaid eggs on the litter floor are more likely to be contaminated and damaged, resulting in economic loss and egg safety concerns. Several management strategies, such as light systems, nest boxes, perches, and robots, have been tested and implemented to control floor eggs. Robots have shown good performance in reducing floor eggs, but these robots lack a detection system to target FELB and non-FELB (NFELB). Therefore, the primary objectives of this research were to develop and test new deep-learning model to detect FELB and evaluate the model’s performance in four research CF facilities using five different YOLOv5 models (YOLOv5n, YOLOv5s, YOLOv5m, YOLOv5l, and YOLOv5x). According to the model performances based on a dataset of around 3000 images, YOLOv5m and YOLOv5x-FELB model size had shown the highest and similar precision (99.9%), recall (99.2%), mAP@50 (99.5%), and F1-score (99.6). However, YOLOv5m-NFELB model sizes resulted in slightly higher precision and lower recall than YOLOv5n, YOLOv5s, YOLOv5l, and YOLOv5x. Thus, the newly developed and trained YOLOv5m model has acceptable in detecting FELB and NFELB in research houses with 720 Hy-Line W-36 hens.
Keywords: machine vision, YOLOv5, floor egg laying, laying hen, cage-free housing
Abstract. In the USA, the primary restaurants or grocers have pledged to buy cage-free (CF) eggs only by 2025 or 2030. However, CF production has several concerns such as floor eggs. Floor eggs have a high chance of contamination by manure resulting in a food safety concern. Manual collection of floor eggs is labor intensive. The objectives of this study were to develop a machine vision method and test the performance in detecting eggs on litter floors timely. The YOLO (You Only Look Once), an advanced object detection technology with very high precision and speed compared to CNN-based algorithms (e.g., R-CNN, Faster RCNN, Mask R-CNN, etc.), was used as a model structure. In this study, we trained “YOLOv5s” network to detect floor eggs of laying hens in research cage-free facilities. Datasets were trained with a batch size of 16 for 200 epochs using Virtual Machine GPU 3.1 provided by Oracle Cloud Infrastructure (OCI) with 6 Oracle CPUs and 90 GB of memory. Results show that the trained algorithm can detect the floor eggs with a precision of 87.9%, recall 86.8%, and mean average precision (mAP) of 90.9 %. Errors were led by image quality as there was dust accumulation on cameras. Cleaning camera frequently can enhance the accuracy of the model.
Keywords: Cage-free system; deep learning; floor eggs; artificial intelligence.
Abstract. Individual egg identification technology has potential applications in breeding, egg production, and anticounterfeiting of high-end brand eggs. This study developed a novel method for its individual identification based on eggshell images. A convolutional neural network-based model, named Eggshell Biometric Identification (EBI) model, was proposed and evaluated. The main workflow included eggshell biometric feature extraction, egg information registration, and egg identification. The image dataset of individual eggshell was first collected from the blunt end region of 770 chicken eggs using an image acquisition platform. The ResNeXt network was then trained as a texture feature extraction module to obtain sufficient eggshell texture features. The EBI model was applied to a test set of 1540 images. The results showed that when an appropriate Euclidean distance threshold for classification was set (17.18), the correct recognition rate and the equal error rate reached 99.96% and 0.02%. This new method provides an efficient and accurate solution for individual egg identification for product tracing and anti-counterfeiting.
Keywords: chicken egg identification, eggshell biometrics, computer vision, convolutional neural networks
Abstract. Successful swine breeding relies on timely and accurate estrus checks of sows and gilts. Estrus checks in current farms are usually performed using the back pressure test (BPT) method, which is labour intensive and inefficient due to the large animal-to-staff ratio. This study aimed to develop a smart sow estrus detection system for stall-housed sows (including gilts). The system consisted of a ceiling-mounted track system, a three dimensional (3D) depth camera, a robotic platform, and an edge-computing system. The edge-computing unit (Raspberry Pi) was used to control the robotic system, connect the camera, and acquire imagery data. Imagery data were acquired at a 10-min interval from the back of sows housed in gestation stalls. Magnetic sensors on the track were used to indicate the position of each stall. Deep learning algorithms implemented on the edge-computing unit were developed to automatically process and analyse infrared (IR) and depth images to quantify sows’ behavioural patterns and vulvar size. Behavioural patterns, including standing and lying, were calculated and used to indicate the onset of estrus. Preliminary study was conducted on 20 sows at a research farm where estrus was checked once a day on the 5th and 6th day after weaning. An estrus detection based on a support vector machine was trained using the daily difference in 20 sows’ vulvar size, daily standing, and daily idle duration as inputs. Results showed that the behavioural patterns could be observed prior to the onset of estrus, such as an increase in daily standing duration and a decrease in daily idle duration. The trained model was validated on six new sows and results showed that overall training accuracy and test accuracy were 95.4% and 93.1%. False alarm for the onset of estrus occurred within one day prior to the detected estrus event. No distinct behaviour pattern was observed around returned estrus. The study indicated that the developed system could be used to help improve sow management efficiency and save labour consumption.
Keywords: swine reproduction, estrus check, robotic imaging system, 3D camera
Abstract. Welfare of sows and piglets receives increasing attention. PLF systems to aid farmers in monitoring pig health and welfare slowly reach the market. Automated behavior monitoring via sound and vision could help farmers to prevent health and welfare issues around farrowing. Five sows were monitored in two field studies. A Sorama L642V sound camera, providing sound source localization through a 64 microphones array, visualizing sound sources as colored spots, and a Bascom XD10-4 security camera with built-in microphone, were used to record vision and sound of sows, piglets and environment around farrowing. In field study 1, sound spots were compared with audible sounds, using The Observer XT (Noldus Information Technology), analyzing video data at normal speed. This gave many false positives, with visible sound spots but no audible sounds. Accuracy was 19.7%, error percentage 80.3, sensitivity 45.3% and specificity 12.8%. During farrowing, 16 from 50 piglet births were visible, but none audible. One piglet was crushed, without any sound. In field study 2, data were analyzed at a 10-fold slower speed and sound spots were compared with audible sounds and sow behaviour. This resulted for audible sounds in 43.6% accuracy, 56.4% error, 100% sensitivity and 9.6% specificity and for sow behavior in 38.8% accuracy, 61.2% error, 100% sensitivity and 8.9% specificity. We conclude that sound cameras are promising tools, detecting sound more accurately than the human ear. There is potential to use sound cameras to detect onset of farrowing, but more research is needed to detect piglet births or crushing.
Keywords: sows, piglets, sound analysis, farrowing, behaviour
Abstract. Establishment of social hierarchy within gestation group housing may lead to increased stress for low ranked sows. The study objective was to evaluate use of nonlinear heart rate (HR) variability as a potential future tool for characterizing social hierarchies. Five groups of sows (≥ 12 sows in each group) were enrolled. One day prior to weaning, baseline HR data were collected from 10 sows in each group for 60 min while in individual farrowing crates. The following day, HR data were collected from the same 10 sows for 240 min after returning to group-housing (reintroduction). Stored HR data were used to calculate sample entropy (SampEn) and short-term detrended fluctuation analysis (DFAα1). Aggressive interactions during reintroduction were used to determine social rank. Sows were classified into one of four rank quartiles (RQ), where RQ1 were high-ranking sows. For baseline, RQ affected SampEn and DFAα1 (P < 0.001 for both), with RQ1 exhibiting greater SampEn (P < 0.01) and a lower DFAα1 value (P < 0.001) compared to all other RQ. Additionally, RQ4 had greater SampEn and DFAα1 than RQ3 (P = 0.02 and 0.03, respectively). No other baseline differences were observed between RQ (P > 0.1). After reintroduction, RQ4 tended to have lower SampEn than RQ3 (P = 0.08), with no other differences between RQ for SampEn or DFAα1 (P > 0.1). Based on these data, baseline SampEn and DFAα1 has potential to predict high-ranking sows in group-housing. Future work needs to investigate other HR measurements as potential predictors of lower-ranked sows.
Keywords: heart rate variability, nonlinear variability analysis, social hierarchy, swine, welfare
Abstract. Objective evaluations conducted at cull sow harvest facilities may serve as an indicator for cull sow health and wellness. These evaluations may include body condition, presence of lesions and abscesses, visceral tissue evaluations, and non-edible trim loss evaluations. Thermal, spatial, and RGB cameras were installed at Midwestern harvest facility to evaluate cull sows immediately prior to their harvest. The recorded images could be used to evaluate welfare indicators in real-time and provide feedback to producers when evaluating sows at harvest. Body condition estimates were obtained using spatial cameras to estimate body weight and indicate condition. These evaluations could assist producers in feed utilization for optimizing cull sow value. Thermal imaging was utilized to evaluate and identify abscesses and sores (hotspots). Utilizing the identified hotspots, their relationship with non-edible trim loss and visceral tissue evaluations was investigated. Skin emissivity, lairage conditions, debris on skin, and other factors are some limitations when utilizing thermal imaging to predict non-edible carcass trim loss. Thermal imaging results revealed that a prevalence for more hotspots in the anterior portion of the carcass resulted in higher non-edible trim amounts. Cumulatively, the application for digital imaging at cull sow harvest facilities may be used to provide information on animal condition that could help producers better understand the sow welfare and condition at harvest. Ongoing work is geared to better assess digital and thermal imaging utilization in evaluating cull sow welfare at the harvest facility and investigate deep learning models on larger data sets having higher spatiotemporal resolution.
Keywords: digital imaging, thermal imaging, body condition score evaluation, trim loss prediction
Abstract. Due to growing concern over the welfare of livestock and changing legislation in the European Union pig farmers will have to adopt new management methods in farrowing pens. An important challenge related to implementation of temporary sow confinement is the optimal timing of confinement in crates, considering the sow welfare and piglet survival. In total, 71 sows and four types of farrowing pens were included in the observational study. To automatically detect the optimal timing of sow confinement we applied computer vision model You Only Look Once X (YOLOX) to detect sows’ locations, calculated activity level of sows’ based on detected locations and detected changes in sows activity trends with Kalman filtering and fixed interval smoothing algorithm. Results indicated the beginning of nest-building behaviour with a median of 12 h 51 min and ending with a median of 2 h 38 min before the beginning of farrowing based on centroids of sows extracted with YOLOX-large object detection model. It was possible to predict farrowing with good performance on validation dataset i.e. for 29 out of 44 sows, considering that the object detection model had to be trained on the farrowing pens present in the validation dataset. The developed method could be applied to warn the farmer when nest-building behaviour starts and then to confine the sow in a crate when the end of nest-building behaviour is detected. This could reduce labour costs otherwise required for regular control of sows in farrowing compartments.
Keywords: sow, nest-building, computer vision, hay rack use, automated monitoring, deep learning
Abstract. Continuous monitoring of the respiratory health status of pigs by means of automated detection of respiratory symptoms offer a solution to treat animals in an early stage of disease development, and consequently reduces the use of antimicrobials. Lately, pig cough classification for respiratory health monitoring using sound has caught the attention of the research community. This study examines the challenges involved in scaling the cough classification solution to newer farms with different acoustical properties. The results show that the models developed on a dataset captured in a certain acoustic condition fail to generalize across different farms with different acoustics. Experiments indicate that the performance can drop by almost 27 percentage points. The usage of a large sound dataset improves to model the realistic variations that are present in the data and avoids having a model bias for a certain acoustic condition. The model performance increases from 80% to 92.4% when samples size is increased from 10,000 to 400,000 samples. We conclude that the size and the acoustical diversity of the sound dataset plays a vital role in achieving a reliable cough classification model.
Keywords: cough analysis, sound event classification, deep learning, transfer learning.
Abstract. Automatically counting and identifying pigs within a facility can improve worker efficiency and ensure proper placement of pigs, though manual identification can lead to misread tags or a miscount of pigs. Ultra-high Frequency (UHF) Radio-Frequency Identification (RFID) could solve this by reading ear tags within 10 m of the antenna, limiting errors such as incorrect identification. Identifying the room location ensures that pigs are correctly located and provides room identification for each pig. The objective of this study was to evaluate the feasibility of a UHF RFID system to identify nursery pigs moving through a doorway. Two groups of nursery pigs were given Electronic Identification (EID) ear tags and RFID readers were placed at three configurations in a hallway. The pigs were guided along the hallway while the RFID system recorded the scanned ear tags and timestamps. Three configurations were tested and compared for differences in accuracy. Reader Position 1 (RP1) was mounted 0.25 m high on the wall and faced perpendicular to the floor. Reader Position 2 (RP2) was 0.66 m high on the wall and angled 45° downward towards the floor, while Reader Position 3 (RP3) was parallel to the floor and mounted 1.07 m high in the center of the aisle. Results showed that RP2 had the greatest accuracy, 54.18%, compared to RP1 and RP3 (48.60% and 20.84%, respectively). This study evaluated the feasibility of the UHF RFID system to detect pig ear tags when moving along a hallway, as well as revealed areas of improvement.
Keywords: counting, inventory, precision livestock farming, location tracking
Abstract. Accurate real-time control is helpful to reduce temperature difference, fluctuation and energy consumption in livestock houses. Studies have shown it is practical significance to forecast and control air temperature accurately. However, the indoor air temperature shows the characteristic of periodic fluctuations and cannot be accurately forecast by traditional models. To forecast data with nonlinear and fluctuate characteristics, a new discrete grey model is proposed in this study, and the predictive data by model as feedback value into the controller to control devices. The proposed model combines time period groups, group-buffered rolling mechanism, and time period group factor to accurately capture the nonlinear and fluctuations in air temperature. Three empirical examples are provided for verifying the efficacy and reliability of the new model by comparing with two existing models. The comparison results indicate that the proposed model generally outperforms existing methods as it can well capture the nonlinear and fluctuating characteristics of temperature.
Keywords: livestock buildings, indoor air temperature, prediction, fluctuations, energy consumption
Abstract. The livestock sector is increasingly using precision livestock farming (PLF) to assist in automated and realtime decision making for management purposes. Among the tools used in PLF, computer vision (CV) is a predominant approach that allows automatic feature extraction from digital images/videos. Thus, CV is useful for monitoring animals and measuring phenotypes. A key to developing CV is training models with annotated imagery data. Unlike general CV, there are relatively few publicly available PLF imagery datasets. Furthermore, despite the potential of CV in PLF, most published CV applications in PLF are developed using small datasets, and their broader validity remains unknown. The goal of this study was to review current public datasets for PLF-CV applications along with the validation strategies used in each related work, as validation is a necessary step to create reference PLF datasets and to develop standard evaluation metrics that can be informative in practical animal farming. We focused on pig and cattle datasets along with their CV tasks. We identified 20 public datasets, nine of which focused on pigs and 11 on cattle. The reviewed datasets span a wide range of CV tasks including detection, behavior recognition, identification, and tracking of animals, which are useful for developing CV algorithms without having to record and annotate new videos. Finally, we provide suggestions to improve image data collection from CV applications in PLF focusing on perspectives of open-science framework for data repositories and suggestions for alternative validation strategies.
Keywords: computer vision, public datasets, validation.
Abstract. The environmental impact of livestock is a topic of growing interest because solutions to reduce it are required. Precision Livestock Farming (PLF) can play a role in this context because PLF tools enable the real time monitoring of animals, determining improved knowledge and capabilities to act efficiently on the herd. This paper deals with a case-study assessment of the environmental impact of a dairy cattle farm located in the South of Italy by adopting the Life Cycle Assessment (LCA) method. Opportunities of improving environmental sustainability on the farm were explored when PLF techniques were used. High temperatures are reached during summer in the geographic region being studied, which can negatively affect the welfare, health, and milk productivity of the cattle on the farm. Incorporating technology for herd monitoring could detect heat stress consequences, enabling mitigation of welfare and production problems and consequently also the environmental impact of the system.
Keywords: real-time recognition, cough analysis, spectral analysis, signal processing
Abstract. Feed activity is becoming economically, technologically, and environmentally important on dairy cow farms. Given that, changes in cows’ feeding behavior may occur due to health disorders, stress, feeding condition, or inadequate diet. Furthermore, it is possible to use animal behavior changes to provide an early indication of diseases. Several authors developed systems using image processing to monitor the animals in real-time and non-invasive ways to measure dairy cows’ behaviors. This study aims to automatically count the time the dairy cows (Holstein) spend at the feeder during the day using image processing. Microsoft Kinect® v2 depth sensors were used for top-view image collection and were positioned above seven feeders for five days. Frames were captured every second. An algorithm was developed in MATLAB® (R2021b) to estimate the time the animal spent in the feeder using the sum of the areas in pixels of the feeders without animals as a threshold. If the area of each feeder was larger than the determined limit, it meant that there was the presence of the animal in the feeder. The total time in seconds was converted to minutes. This method was compared with the manual labeling of the images. Preliminary results show that it was possible to estimate the time of animals’ spent in the feeders during the five days of monitoring through image processing, with an accuracy of 94.71% and an error of 5.28%. The results indicate that the developed model can effectively and practically estimate feeding behavior management during dairy production.
Keywords: depth sensor, image processing, dairy cows’ behavior
Abstract. The daily activity and suckling behavior of eight lambs in the first three weeks of life was measured by actigraphy. Lambs were artificially fed reconstituted milk replacer through an automatic lamb feeder. To quantify proximity to the milk feeders, the lambs were fitted collars that held accelerometers that were Bluetooth-enabled (wGT3X-BT; ActiGraph; FL, USA). The sensors on the lambs were programmed as beacons, and two sensors that were installed close to the rubber nipples of the feeder were programmed as receivers, which recorded the signals from other beacons (lambs) nearby. The activity data were used to calculate the Vector Magnitude (VM). Mean (±SE) VM was 140±3 counts/min (daytime 180±6; nighttime 107±3; P<0.001), and activity was significantly (P<0.001) higher in week 2 than it was in week 1 and week 3. Overall VM did not differ significantly between males (139±5) and females (142±5). Mean number of meals/day/lamb was 3.7±0.2 (12.5±0.9 min suckling/week, 3.5±0.2 min/meal). The fewest number of meals/day and the lowest total min/week occurred in week 3 (P<0.05), and the number of min/meal did not differ significantly among weeks. Males and females did not differ significantly in the number of meals/day (males: 3.8±0.3; females: 3.4±0.3) or in the time spent suckling (males: 14.9±1.5; females: 10.1±0.8 min/week); however, males (4.1±0.4 min) dedicated more time/meal than did females (3.0±0.2 min) (P<0.05). In conclusion, actigraphy can be useful for measuring the behavior of lambs reared artificially and, thereby, improve this particular management of lambs.
Keywords: actigraphy, lambs, artificial rearing, activity
Abstract. Hoof care is an important aspect of sheep management, particularly, in intensive systems where the need for hoof trimming is most likely to occur because animals do not move around extensively. Hoof ailments can affect welfare and productivity negatively because overgrown hooves make walking difficult and predispose the animal to other foot problems. Ten adult ewes were used to quantify the effects of hoof trimming on their welfare as measured by activity rate after excessive hoof growth had occurred. Animals were assigned to one of two groups; ewes that had not had hoof trimming in the previous eight months [overgrown hooves (OH), n=5] and ewes that had normal hooves (NH, n=5) and had had the last trimming four months earlier. One week before and one week after hoof trimming, ewes were fitted with sensors that record high resolution raw acceleration data (wGT3X-BT; ActiGraph, FL), which were attached to a neck collar. The activity data (activity counts/min) were transformed into Vector Magnitude (VM). The two groups differed significantly in mean (±SEM) daily VM the week before (OH: 47.2±4.7; NH: 65.1±3.1 counts/min; P<0.05) and the week after hoof trimming (OH: 34.4±3.5; NH: 61.4±3.5 counts/min; P<0.01); i.e., OH ewes were always less active than were NH ewes. Furthermore, hoof trimming significantly (P<0.01) reduced the activity of the OH ewes. In conclusion, allowing excessive growth of the hooves reduced the welfare of the sheep as reflected by reduced activity, which did not improve after hoof trimming.
Keywords: actigraphy, sheep, hoof trimming, welfare
Abstract. Rams exposed to two months of artificial long days in winter followed by the natural photoperiod have increased testosterone concentrations and improved sexual performance in the seasonal anestrus. This study used actigraphy to quantify the locomotor and circadian behavior of sexually activated rams in spring. To confirm 24-h circadian rhythmicity, an F-test (p<0.05) was used to compare the cosine model and the nonrhythmic model. Sixteen adult rams were exposed to either an artificial photoperiod (16 h light/day, 15 Dec- 15 Feb) (AP, n=8) or the natural photoperiod (NP, n=8). At the end of the long days, AP rams were returned to the natural photoperiod. Forty-five days after the end of the long-day exposure (1 Apr), rams were fitted with sensors that record high resolution raw acceleration data (wGT3X-BT; ActiGraph, FL) attached to a neck collar for one week. The activity data (activity counts/min) were transformed into Vector Magnitude (VM). Mean (±SEM) VM was significantly (P<0.05) higher in AP rams (66.0±3.3) than it was in NP rams (57.5±2.8), especially in the day (0800-2000 h) (AP = 93.9±4.0, NP = 81.1±3.6, P<0.05; night: AP = 32.9±2.3, NP = 29.7±1.9, P>0.05). All eight AP rams exhibited a 24-h circadian rhythm, but only 37.5% (3/8) of the NP rams did so (P<0.05). In conclusion, if sexually activated rams are more active than are non-active, anestrous rams, actigraphy might be useful for detecting and choosing the most effective rams in the flock in the mating season.
Keywords: actigraphy, rams, light, activity
Abstract. During 7 days, the estrus of three nulliparous (NP) and three multiparous (MP) ewes was hormonallysynchronized using a protocol based on vaginal sponges impregnated with progestogen, and 1 ml Prostaglandin-F2α and 2 ml PMSG at sponge withdrawal. At that moment, a surgically implanted subcutaneous bio-logger (DST milli-HRT, Star Oddi, Iceland) (13×39.5 mm, 12 g) was placed in each ewe, programmed to record body temperature (T) and heart rate (HR) every five minutes for seven days after sponge removal. Two days after the biosensor placement, a ram was introduced in each group of ewes. NP ewes had lower T (38.59±0.68 vs. 38.80±0.71ºC; p<0.01) and a higher HR (97.94±42.21 vs. 84.72±26.50 bpm; p<0.01) than MP ewes. Furthermore, in both groups of ewes, the lowest T (NP: 38.03±0.63; MP: 38.01±0.94ºC) and HR (NP: 87.08±40.66; MP: 75.84±24.72 bpm) were recorded in the two days after the removal of the sponges, when the animals were still under the effects of the hormonal treatment. The highest T (NP: 38.92±0.91; MP: 39.03±0.48ºC) were recorded during the fourth day post-hormone administration, while the highest HR (NP: 104.66±43.65; MP:88.25±25.33 bpm MP) was recorded during the third day after the removal of the sponges, coinciding with the observable heat of the ewes. Overall, these bio-loggers revealed physiological variations of ewes caused by hormonal treatments used for estrous synchronization and by the estrus itself. This might be very useful to predict the optimal moment for mating, which could be even more interesting in the case of artificial insemination.
Keywords: bio-loggers, sheep, temperature, heart rate, estrus
Abstract. LoRa-WAN sensors were used to compare methods for determining walking distances by grazing cattle in near real-time. The accuracy of relying on a global positioning system (GPS) alone or in combination with motion data derived from triaxial accelerometers was compared using stationary control trackers (Control) placed in fixed field locations (n=6) vs. trackers (Animal) mounted on cows (n=6) grazing on pasture at the New Mexico State University’s Clayton Livestock Research Center. Trackers communicated motion data at 1- minute intervals and GPS positions at 15-minute intervals for seven days. Daily distance walked was determined using: 1) raw GPS data (RawDist), 2) data with erroneous GPS locations removed (CorrectedDist), or 3) data with erroneous GPS locations removed and with GPS data associated with the static state excluded (CorrectedDist_Act). Distances were analyzed via one-way ANOVA to compare Control vs. Animal deployment effects. No difference (P=0.43) in walking distance was detected between Control vs. Animal for RawDist. However, distances calculated for CorrectedDist differed (P<0.01) between the two tracker deployments. Due to the random error of GPS measurements, CorrectedDist for stationary devices differed (P=0.01) from zero. The walking distance calculated by CorrectedDist_Act differed (P<0.01) between Control vs. Animal trackers, with distances for Control trackers not differing (P=0.44) from zero. The fusion of GPS and accelerometer data was a more suitable method for calculating walking distance by grazing cattle. This result may highlight the value of combining more than one source of independent sensor data in Precision Livestock Farming applications.
Keywords: Precision Livestock Farming, precision livestock ranching, Internet of Things, long range wide area network
Abstract. Behaviour analysis is used to assess the welfare of animals to different environmental stimuli. Recording behavioural data is an arduous and repetitive task for humans. The present research aimed to develop a multi-tracking system that individually monitors laying hens. The proof of concept was carried out with a 30- minute video experiment with six laying hens, housed in an Environmental Preference Chamber (EPC), with three compartments interconnected that allowed the birds free choice. Each compartment had a surveillance camera installed on the ceiling, which were later joined by the passages, generating the 30 min video. A YOLOv4 network was trained to detect the hens and provide the coordinates in the frame. These coordinates composed the input of a multi-tracking system based on a Kalman filter, which tracked each bird in the three compartments simultaneously, without losing the identification of each bird. The system recorded the time and distance travelled by each hen in each room, with an accuracy of 99% for the detection of birds. We observed the occurrence of few identifications’ maintenance errors during tracking, originated from the failure to detect of the hens by the YOLO the concealment of the birds in the nests. In these cases, the multi-tracking system generates a new identifier for the same bird. We consider that for this study the multi-tracking system developed was efficient to detect and maintain the identification of birds during monitoring. The observed errors can be minimized by improving the algorithm.
Keywords: computer vision, YOLO, deep learning
Abstract. Virtual fencing (VF) is an alternative method to control livestock dispersal. This method consists of the use of animal wearable collars that employ auditory-electric pulse cues to deter animals from exiting their predefined containment zones. The study aimed to document skin defense (SkinM) and association learning mechanism (AssocM) in describing the conditioning behavior of the VF application. Nursing Brangus cows at the New Mexico State University’s Chihuahuan Desert Rangeland Research Center were allotted three days of free access to feeding areas (0.19ha) with VF-deactivated (VF-Off) or VF-Activated (VF-On) collars restricting one-third of the penned area. This training sequence was repeated twice (6-day/Period) with two replications (n=11 and 17cows). The VF collars communicated real-time animal positions at 15-minute intervals. ANOVA was used to compare daily-derived variables per cattle on the percentage of time spent within the containment and restricted zones (SkinM) and the number of auditory and electric pulses emitted during the VF-On configurations (AssocM). The VF-On treatment increased the percentage of time collared animals spent within the containment zone (98.4 vs.72.0 ±1.0 %Time;P<0.01) and reduced the percentage of time within the restricted zone (1.6 vs.28.0 ±1.0 %Time;P<0.01) compared to the VF-Off treatment. Exposure to VFOn in Period 1 triggered a greater frequency of auditory (1.8 vs.0.6 ±0.4;P<0.01) and electrical pulses (0.7 vs.0.2 ±0.2;P<0.01) than in Period 2. Results indicate that groups of cows learn rapidly to respond to VF boundaries by reducing the time spent within the restricted areas (SkinM) and relying increasingly on auditory cues to alter behavior (AssocM).
Keywords: animal tracking, digital ranching, precision grazing, virtual herding, virtual fence
Abstract. Over 90% of pigs internationally are bred by artificial insemination (AI). The most critical component of successful AI breeding is a reliable insemination schedule facilitating high conception rates, high farrowing rates, and large litter sizes. Inaccurate insemination timing from missed estrus events is a major contributor to AI failure and can result in losses of time, money, and reproductive output (Lamberson and Safranski, 2000). The need for specialized labor to conduct estrus detection checks also places undue burdens on the already limited supply of farm labor. The objectives of this paper are to demonstrate the potential for an electronic capture system to independently identify specific pig postures and extrapolate how this approach can be utilized in the estrus detection process. Our remote YOLO v4 model detected slight increases in time spent in standing postures in a small sample of gilts around the time of estrus. This may lay the groundwork for expanded estrus detection technology that could increase daily farm efficiency, standardize evaluations of estrus detection, and lessen the need for specialized human labor to conduct estrus detection checks.
Keywords: artificial intelligence, machine learning
Abstract. The behavior is the bird’s first response to an adverse environment capable of providing an indicator of wellbeing in real-time. The combination of behavioral parameters with the use of automated techniques improves the decision-making in poultry farms. The use of the sequential pattern mining technique applied to a broiler behavior dataset provides specific sequential patterns of behavior under conditions of different temperatures. The behavior dataset of broiler chickens aged 21 to 42 days was analyzed under thermoneutral and stressful temperatures. The Generalized Sequential Pattern Algorithm (GSP) analyzed the behavior database. The results indicated: (1) through the GSP algorithm it is possible to predict behavior patterns in the different variations evaluated from the behavioral sequences in the growing and finishing phases; (2) broilers exposed to temperatures at 8 °C both above and below thermoneutral conditions tend to decrease locomotor activities (3) short behavioral sequences were characteristic of heat stress; (4) behavior that is considered characteristic of heat stress, only appeared in the sequential behavioral patterns of chickens under heat stress; (5) sequence pattern mining is a valuable and simple technique to estimate the welfare of broilers, allowing the identification of temporal relationships between heat stress and consequent behavior of the broiler; (6) the welfare of broilers can be estimated through the length of the behavior sequences; (7) we were able to identify temporal relationships between heat stress and behavior of broiler, reassuring the need for further studies on the use of temporal behavior sequences in environmental controllers.
Abstract. This study analyzed the effects of locking plate fixation used for bridging of tibial segmental ostectomy and of cast immobilization on goat gait biometrics. We hypothesized that stable fixation of a segmental bone defect, using a locking plate construct, would result in minimal changes in biometric variables of gait in goats, but full-limb immobilization would result in lasting alterations in the immobilized limb’s gait kinetics. A pressure-sensing walkway was used to measure biometric characteristics for stride, gait, and walking force. Thirteen, non-lame adult Boer-cross goats were trained to walk over a pressure-sensing walkway prior to instrumentation. Segmental ostectomy was performed on the right hind tibia of each goat and the defect was stabilized locking plate fixation. The goats underwent right hindlimb cast immobilization for a period of one to three months after the surgical procedure. Data was collected preoperatively and then over twelve months postoperatively in goats with unrestricted mobility. Statistical analysis revealed no significant alterations in hindlimb kinematics or maximum force between surgery and cast immobilization. However, significant decreases in forelimb stride length and velocity were noted postoperatively but normalized prior to cast placement, suggesting the overall functional stability of fixation. Cast immobilization had a profound and sustained effect on gait with significant alterations in both forelimb kinetics and hindlimb kinetics and kinematics for the remainder of the trial period. Increased hindlimb asymmetry characterized by greater weight distribution and impulse to the left hindlimb was observed, suggesting the potential for long-term and/or permanent detrimental effects of prolonged limb immobilization.
Abstract. Currently, accurate quantification of tick infestation levels for effective selection of tick tolerant cattle, remains a global challenge. This study explored the convolutional neural networks algorithms for detection and quantification of tick burdens on cattle. The present study aimed to evaluate the efficacy of convolution neural networks in detection and quantification of tick burdens on cattle as an alternative for conventional visual methods. It is hypothesized that application of CNNs on the algorithms can detect and quantify ticks in cattle. The deep learning algorithms with architectures: “ConvNet ” and “MobileNet” were trained on a dataset of 1124 “thermograms” to detect ticks on cattle. The algorithms were evaluated and validated for rapid and accurate tick detection. The transformation of images during training was observed. ConvNet model achieved a training and validation accuracy of ~90 and 60%, respectively. Whereas MobileNet scored a training and validation accuracy of ~95 and 75%, respectively. For MobileNet, data augmentation and feature extractor layers were rescaled using Keras Functional API to build a model. After evaluation, the model was then ready to predict the number of ticks in a thermogram. Deep learning was successfully used to detect ticks on cattle using pre-trained CNNs, which facilitates tick control and selection of tick tolerant animals. It is recommended to use camera with better resolution and sensitivity and capturing images aiming at maximizing the contrast between ticks and the host animal to obtain higher accuracies when training the algorithm(s).
Abstract. Automated sensor data has incredible potential to provide actionable management information to dairy producers. Wearable sensors were developed to monitor individual animal behavior related to health and production. We hypothesized that sensor measurements may be associated with feed intake and other feed efficiency traits. Four different wearable technologies were evaluated, including: rumen bolus (1), ear tags (2), and milking collar (1) data with n=56–676 lactating Holstein cows, in parities 1-6 ranging from 24-278 Days in Milk (DIM). Daily milk yield and feed intake measured for 40-74d, weekly milk fat, protein and lactose and body weight and condition score (BCS) measurements were included for analyses. Individuals with health events were excluded. Statistical associations with dry mater intake (DMI) were evaluated in PROC MIXED of SAS, accounting for effects of contemporary/season, parity, DIM and energy sink variables (i.e., milk yield, fat, protein, lactose, BCS, metabolic body weight = BW0.75) known to impact cow feed efficiency. Sensor measurements included: activity (3), rumination (1), ear temperature (1), rumen pH (1), and temperature (1). Significant associations (p<0.05) with DMI were identified with nearly all measurements with the largest estimate for rumen temperature (-3.48 ± 0.32kg/°C; p<0.0001). Associations of feed intake with activity were identified across all sensor devices (p<0.05). Notably, all measurements were able to account for additional model variability in DMI beyond the variables routinely included in feed efficiency estimates. Though validation is required, wearable sensor data appear to provide new information that may help more accurately identify variation in individual cow feed intake.
Abstract. Cellulose paper, as a non-toxic, environmentally friendly, and renewable material, has low price, simple preparation and mass production, which is suitable for the substrate of field-effect biosensor (BioFET). However, its inherent moisture absorption causes swelling and contamination in serum, affecting the stability, sensitivity, and service life of BioFET; besides, its bad corrosion resistance in organic solvent increases the difficulty of modification. In this study, a micro- to nanoscale hydrophobic material was synthesized using octadecyltrichlorosilane, which were further decorated on cellulose paper with outstanding surperhydrophobicity. The superhydrophobic paper was applied as BioFET’s substrate, significantly enhancing stability and service life. In addition, organic silica gel instead of wax was constructed the BioFET’s barrier(SPFET) with high corrosion resistance to decrease the difficulty of modification. And pyrene carboxylic acid-modified semiconducting single-walled carbon nanotube(SWNTs-PCA) in organic solvent could decorate the BioFET with no need of further treatment. Two DNAzymes with different sensitivity was designed by changing sequence length and cleavage site, and functionalized with SPFET/SWNTs-PCA to formed Dual-BioFET, decreasing the interference of impurities in serum. After optimization, Dual-BioFET can detect the Ca2+ concentration in the range from 25 μM to 5 mM with a detection limit of 10.7 μM, which can meet the requirements of hypocalcemia diagnosis.
Abstract. The body of work involving computer vision and pigs is difficult to build on previous efforts because the availability of robust datasets is limited. Most computer vision efforts in applications for pigs have utilized custom datasets for individual challenges and specialized cases. An open-access benchmark dataset that encompasses pig production system complexities could attract cutting edge development in new computer vision and artificial intelligence techniques. We applied a custom animal behavior analysis tool (AVAT) to create a general-purpose dataset (PigLife) that contains video clips and images across most pig production phases in commercial systems: Breeding & Gestation, Farrow to Wean, Nursery, and Grow-Finish. We applied the AVAT to label every frame of the PigLife dataset with individual identification, instance segmentation, posture labels (4 classes), behavior labels (7 classes), and occlusion labels (5 classes). The PigLife dataset has been created to support benchmarking or model development. The dataset consists of 40 short video clips (5 seconds~2 minutes) containing scenarios of a single pig, multiple pigs (up to 17 pigs), and farrowing sow with a group of piglets. Each pig production phase includes at least three types of vision and environment variation (view angle, lighting condition, housing type, etc.). The framework for hosting the dataset has been created to accommodate further expansions of this dataset and with the opportunity to host additional datasets with common standards for formatting and sharing. We present a detailed description of the dataset and demonstrate its multi-task application (detection, segmentation, identification) and how to use metadata for error analysis.
Abstract. Herd monitoring through image analysis can be performed at either an individual or group level. Animal identification has become necessary to enable individual animal monitoring. The most commonly used method of livestock identification is via passive Radio Frequency Identification (RFID) tags. These low-cost tags are commonly pierced on the animals’ ears in a time-consuming and stressful process. They have a limited range at which they can be activated and read successfully, and multiple tags cannot be read concomitantly. Common elements in the farm environment made of metal can also be detrimental to the antenna’s effectiveness and further reduce the range of reading or prevent it. With those factors, it is not trivial to conclude that a more efficient and less invasive animal identification method that still enables realtime data analysis has become necessary. Image processing and computer vision could be the solution. This study attempts to produce a model for swine recognition that can not only distinguish between individual pigs but also adapt and continue to recognize growing pigs as the same individual over time.
Abstract. The purpose of this study was to develop a thermal balance model to allow for the prediction of dynamic responses of a dairy cow to high temperature (heat stress) conditions. The model is based on previous models and was improved using recent experimental data to allow animal responses to be adjusted at the same time to given environmental conditions. Tissue resistance, respiration rate and sweating rate were related to skin temperature. Metabolic heat production was related to characteristics of cows as well as to air temperature. Model inputs include cow body weight, daily milk production, pregnant days, dry-bulb temperature, relative humidity and air velocity. The model included three nodes (body core, skin and coat) and therefore three differential equations were developed to represent the dynamic heat change based on the energy balances of each node. The model was evaluated with experimental data measured on 20 cows from climate-controlled respiration chambers. The root mean squared error of prediction was 0.3°C for body temperature and 1.2°C for skin temperature, respectively. The model was likely to overestimate the body temperature, given some predictions were higher than the observations with mean bias of -0.11°C. The model gave higher values of body and skin temperatures when cows were exposed to the same warm conditions for a duration of 8 hours compared to a duration of 1 hour, which was in agreement with experimental findings. Besides, the benefits and limitations of different cooling methods were predicted under various environmental conditions.
Abstract. The body weight of broiler chicken is a crucial indicator for productivity and animal welfare. Accurate, continuous and rapid estimation of broiler body weight can effectively reduce the workload of farmers and better track the growth status and performance of broilers, which can be achieved via developing and applying precision livestock farming technologies. The objective of this study is to develop a multi-view depth camera vision system for estimating body weight parameters of individual broilers in real time. Three depth cameras were used to capture images of individual broilers from three directions (i.e., top view, two side views). Based on the depth data, the broiler volume was calculated using a numerical integration method. A back propagation (BP) neural network prediction model was developed to predict body weight using the parameters of bird’s back width, body width, body height and volume. The results showed that the prediction model could predict the body weight of broilers well, with the coefficient of variation and relative error within 5% and 10%, respectively. The system can be used as a prototype of vision-based method to measure the body weight of broilers and provide an automated solution for measuring bird body weight in broiler production industry.
Abstract. Voluntary soaking systems for heat stress abatement provides the cow freedom of choice and the opportunity to self-manage heat stress. Hence, this precision dairy technology (PDT) targets the individual cow needs and provides the animal with agency. We have developed and validated a voluntary radiofrequency controlled soaking system capable of identifying cows automatically and providing them with a 10 second soaking cycle at 30L/min. in our prior study, there was great individual variation in voluntary soaker use ranging from 0 to 227 soakings/d, (13±30; mean ± SD). Thus, the objective of our project was to determine the effects of the voluntary soaking system on the behavior, physiology, and production of dairy cows milked in voluntary milking systems. During the study, the voluntary radio-frequency controlled soaking system operates in three commercial dairy farms with voluntary milking systems (VMS). Following our previous study, for two weeks, cows are trained to use the voluntary soaker by guiding them to the system sporadically throughout the day. At enrollment, animals are randomly assigned to either have unlimited voluntary soakings or no voluntary soakings by crossover design. Each treatment period of 21 days in duration (14 days for treatment adaptation and 7 days of data collection). Treatments are balanced by lactation number, milk yield, and body condition score. We hypothesize that cows with unlimited access to the voluntary soaking system will have lower respiration rates, lower panting scores, lower dehydration scores, and greater milk yield when compared to cows without access to the voluntary soaking system.
Abstract. In recent years, the livestock breeding industry is being converted into large-scale, intensive and intelligentize production mode. The Internet of things (IoT) is under rapid development, which promotes the development of smart agriculture. The improvement of agricultural IoT is also driving the development of precision livestock farming. Based on the structure of IoT, an intelligent service platform of facility environment is built. The platform consists of four layers including information awareness layer, network layer, management service layer and application layer. Through using the distributed network architecture, asynchronous data transmission and the distributed file system, the platform can centrally manage multiple farms data. The functions are included in the platform as accessing and displaying the internal and external environmental data and water and electricity consumption of livestock house, performing data analysis, managing production data as well as the basic data of farms, and managing the system. The service platform was applied in an actual layer house.
Abstract. The accurate and rapid detection of objects in videos facilitates the identification of abnormal behaviors in pigs and the introduction of preventive measures to reduce morbidity. In addition, accurate and effective pig detection algorithms provide a basis for pig behavior analysis and management decision-making. Monitoring the posture of pigs can enable detection of the precursors of pig diseases in a timely manner and identify factors that impact pigs’ health, which helps to evaluate their health status and comfort. Excessive sitting represents abnormal behavior when pigs are frustrated in a restricted environment. The main contributions of this paper are as follows: a human-annotated dataset of standing, lying, and sitting postures captured by 2D cameras during the day and night in a pig barn was established, and a simplified copy, paste, and label smoothing strategy was applied to solve the problem of class imbalance caused by the lack of sitting postures among pigs in the dataset. The improved YOLOX has an AP0.5 of 99.5% and AP0.5–0.95 of 91% in pig position detection; an AP0.5 of 90.9% and AP0.5–0.95 of 82.8% in sitting posture recognition; and an mAP0.5 of 95.7% and mAP0.5–0.95 of 87.2% in all posture recognition. The method proposed in our study can improve the position detection and posture recognition of grouped pigs effectively, especially for pig sitting posture recognition, and can meet the needs of practical application in pig farms.
Abstract. Thermal environment impacts livestock welfare and productivity. Animals’ behavior patterns are altered by high temperatures; however, little is known about how behavioral shifts can be moderated or how they contribute to productivity declines. The use of wearable inertial measurement unit (IMU) sensors has increased the feasibility of behavioral monitoring in livestock due to the success of these sensors at classifying behaviors; however, verification of IMU-based behavior classification during heat stress is incomplete. Our objective was to use an open-source IMU to explore animal behavior classifications under different temperatures. Four crossbred sheep were fitted with an IMU sensor comprised of a generic MPU- 9250 9 degree of freedom IMU, an Arduino-compatible microprocessor, and a data storage module. Animals were assigned to 1 of 2 groups to 1 of 2 rooms where they were housed for 20 d before switching rooms. The thermal environment was changed five times (every 4 d) starting at thermoneutral (20°C, 27°C, 35°C, 27°C, 20°C) in one room and hot (35°C, 27°C, 20°C, 27°C, 35°C) in the other room. Four behaviors (eating, lying, standing, and ruminating) were recorded through visual observation. A random forest regression was capable of distinguishing eating, lying, and lying and ruminating behaviors with similar accuracy (~76%), and similar, but lower accuracy for standing and standing and ruminating (67%). These accuracies are lower than observed in previous studies conducted in thermoneutral environments suggesting that additional data collection and verification efforts are needed to ensure IMU sensing can be leveraged in heat-stressed conditions.
Abstract. Heart rate (HR) measurement can be used as a physiological indicator of heat stress in livestock; however, monitoring HR in animals remains a challenge and requires animal restraint. Photoplethysmography (PPG) sensing has been proposed as an alternative, non-invasive tool for HR monitoring. The purpose of this work was to evaluate the precision and accuracy of a PPG sensor in monitoring HR in sheep experiencing heat stress. Four crossbred sheep were divided into 1 of 2 groups exposed to different thermal environments changing every 4 days. The first group was first exposed to a low temperature (20°C, 27°C, 35°C, 27°C, 20°C) and the second group was first exposed to a high temperature (35°C, 27°C, 20°C, 27°C, 35°C). Manual heart rates (ground truth observations) were collected via stethoscope twice daily and automatically (sensed observations) by an open-source sensor comprised of a microprocessor (SparkFun ® ThingPlus) and a PPG (SparkFun ® MAX30101 & MAX32664) placed on a neck collar. Pearson correlation, root means squared prediction errors (RMSE), and a random forest regression were used to explore precision, accuracy, and HR classification, respectively. With a negative correlation (-0.23), an RMSE of 36.41 %, and classification accuracies ranging from 40 to 55%, we concluded that PPG sensors were ineffective on measuring HR in sheep and future work should focus on securing the sensor more effectively and leveraging data analytics to reduce noise within the measurement.
Abstract. Manually observing individual chickens in commercial broiler production housing systems has become a tedious and demanding job. Vision-based crowd counting and tracking systems using advanced computer vision and machine learning methods have gained popularity over the years to locate subjects and analyze location-based data for behavioral research automatically. Following this trend, automated crowd counting and tracking have become very important in quickly identifying individual chickens in large groups for populational and behavioral analysis. However, in real-world scenarios, fully supervised deep learning methods usually learn to predict through a training process that requires an extensive annotation of densely populated subjects in thousands of images. Directly employing deep learning models that are trained on existing datasets to a new dataset suffers from a significant performance decrease due to the domain gap. Therefore, we introduce a new training approach to the crowd-counting task toward a domain adaptation setting where the deep learning algorithm utilizes entropy minimization and adversarial learning to alleviate the distributional discrepancy between the source domain and the target domain. While the network is trained with labelled data, samples without labels from the target domain are also added to the training process. In this process, the entropy map is computed and minimized in addition to the adversarial training process designed in parallel. Experiments on Shanghaitech, UCF_CC_50, and UCF-QNRF datasets prove a more generalized improvement of our method over the other state-of-the-art in the cross-domain setting.
Abstract. Facial expression is important information about expressed emotion. It is a comprehensive reflection of livestock physiology, psychology, and behavior. It can be used to evaluate livestock welfare. Therefore, the successful recognition of animal expressions is of great significance in the field of animal welfare. We propose a joint Multi-attention Cascaded LSTM model (MA-LSTM) framework to recognize pig’s temporal facial expressions in our work. Firstly, a simplified multi-task convolution neural network is used to detect and locate the pig face in the frame image. Secondly, a multi-attention mechanism is proposed, which makes use of the characteristics that different feature channels have different visual information and corresponding peak response regions are also different. By clustering the regions with similar peak responses, the facial salient regions caused by facial expression changes are captured. Facial salient regions are used to focus on subtle changes in the pig face. Finally, the convolution feature and attention feature are fused and input into LSTM to classify the data. The proposed method can effectively extract the key area features of the pig face required by the network according to the difference in animal expressions, and ensure the timing of pig face expression recognition at the same time. To validate the effectiveness of our proposed model, we work with technicians to collect an expression dataset from a pig breeding enterprise in Nong’an County, Jilin Province, China.
Abstract. The safety and welfare of horses and riders is of utmost importance to the equine community, especially in those disciplines which are high-risk, like jumping. Wearable technologies leveraging inertial motion sensing coupled with GPS have shown promise at evaluating equine biomechanics to provide quantitative measurements of jump quality, which if sensitive enough, could help provide real-time feedback to riders to facilitate improved horse health and performance. Our objective was to evaluate sensitivity of sensed biomechanical measurements to variation in horse and rider experience level. Participants were solicited on a volunteer basis through an email-based referral request. A total of 6 farms participated, allowing data collection on 29 horses 22 riders, resulting in a total of 24 total pairings with usable data. Horses and riders were categorized into “Beginner”, “Intermediate”, or “Advanced” experience levels based on previous competition history. Each horse was equipped with a sensor which was affixed centrally to the girth, and data were collected for the duration of a regular training session. Data were analyzed using linear mixedeffects model with breed, farm, and weather conditions as random effects. Horse and rider experience level and their interaction, jump height, and jump type were considered fixed effects. Nearly all biomechanical factors were affected by horse and rider experience level or the interaction of these factors, suggesting that inertial sensing coupled with GPS may be a useful tool for more quantitatively benchmark horse jump parameters toward the goal of enhancing sporthorse welfare.
Abstract. Photoplethysmography (PPG) sensing is used to measure heart rate and oxygen saturation in humans. Despite success in the human health industry, there has been limited PPG sensor testing in the equine industry, and the translatability of these sensors to horses is unknown. The purpose of this study was to evaluate the accuracy of wearable PPG sensors, originally designed for humans, in monitoring distal limb pulse of horses. The study utilized six mature (9 to 22 years of age) horses of various breeds and sexes (5 geldings, 1 mare). Data were collected by placing a modified exercise boot containing a PPG sensor on the distal limb. Each horse was subjected to 80 readings: 20 per limb with 10 on each side of the limb. Heart rates were taken manually with a stethoscope prior to each recording. To assess sensor accuracy, the Root Mean Square Prediction Error (RMSPE) of raw values was calculated, RMSPE within limb and location were also calculated for each horse. A general linear model with fixed effects for limb and location and random effect for horse was used to evaluate agreement between measured and sensed data and to evaluate the influence of device placement on accuracy. Results indicated that the PPG-based sensor was ineffective at measuring horse heart rates accurately, irrespective of location; however, it was accurate in some horses and extremely inaccurate in others. Future work around designing effective PPG sensors for horses should evaluate this horse-to-horse variation to better understand casual factors.
Abstract. Respiratory diseases are a significant cause of mortality and decreased productivity in pigs. In 2018, the African Swine Fever pandemic resulted in 43.46 million pigs killed either from the disease itself or from culling required to prevent disease spread. This yielded a total economic loss of 0.78% of China’s GDP in 2019. In light of this recent catastrophe, disease surveillance towards early prevention has been brought to the forefront of precision livestock farming. However, frequent manual checking of pig cough occurrence is labor-intense and difficult to manage especially on larger farms. An automatic pig cough monitoring device that operates ig coughs 24/7 is, therefore, a valuable tool for the farm manager. In this paper, we detail an end-to-end solution from detection to the communication of surveillance to the farm manager; the development of which requires a multi-disciplinary skillset spanning from hardware to software. In particular, our solution begins with a TinyML edge-based artificial intelligence-based detection algorithm, allowing for an objective measure of pig cough occurrence and classification throughout the farm. This is followed by an IoT ecosystem that ensures robust communication of surveillance throughout the farm and to a specified endpoint that is in reach of the farm manager. At this endpoint is a dashboard that distils and presents this information in a convenient and informative manner.
Abstract. Climate change causes a higher risk of heat stress (HS) in cows, even in moderate-climate countries. HS has consequences on production and health. Early detection of HS can help a farmer intervene and thus limit the negative consequences. This research assessed the effect of different risk levels for developing HS (low (LR), mild (MR) and high (HR)) on activity when cows are kept indoors year-round in a naturally ventilated barn. Commercial accelerometers on the neck and leg (SmartTag, Nedap Livestock Management) measured lying, eating and ruminating on a farm with 130 dairy cows. Temperature and relative humidity were measured and the THI was calculated. Based on average THI, three periods, of three or five consecutive days, were defined: LR (THI<68), MR (68≤THI≤75) and HR (THI>75). Effect on the behaviors was analyzed with an ANOVA with risk level (LR, MR, HR), parity (≤2;>2) and lactation days (<100;100-200;>200) as independent variables. Lying decreased from 736 (LR) and 749 (MR) to 629 minutes/day (HR, P<0.05). Rumination decreased from 569 (LR) and 577 (MR) to 503 minutes/day (HR, P<0.05). Eating increased from 253 (LR) to 289 minutes/day (MR, P<0.05) and decreased to 137 minutes/day (HR, P<0.05). In conclusion, all behaviors decreased in the HR compared to LR and MR. No changes were found in behaviors in the MR compared to the LR except for an increase in eating time. This increase is unexpected and needs more research to study whether this is a repeatable change and might be an early indicator of HS.
Keynote Speakers
Topics
Sensors and Sensing in PLF
- Development of sensor systems for animal and animal facilities
- Implementation of sensor systems
- Sensing technologies for high-throughput phenotyping
- Robotics in animal facilities
Data Management and Algorithm Development
- Artificial Intelligence (AI) solutions for animal monitoring and management
- Internet of Things (loT) applications for data management
- Analysis on multitype data (e.g., image, video, sound, etc.)
- Data security
Measuring, Modeling, and Managing of Dynamic Responses
- Real-time animal welfare and behavior monitoring
- Advanced modeling techniques in PLF
- PLF for detecting animal disease
- Precision animal environment and nutrition management
- PLF solutions for management decision-making
- Case studies of PLF field applications
Societal Impacts of PLF
- Economics of PLF systems
- Ethics in PLF
- Consumer and farmer perceptions on PLF
- Challenges and opportunities for PLF
- Sustainable animal production
Commercial PLF Systems and Field Application Experience – Open for farmers and PLF system providers with abstract submission (full paper is not required)
Key Deadlines
Abstract submission opening: May 15, 2022
Abstract submission closing: July 31, 2022
Invitation to submit full paper: August 31, 2022
Full paper submission due: January 15, 2023
Review feedback to authors: March 15, 2023
Final full paper submission due: April 15, 2023
Field Tours
UTIA Robotic Dairy Farm, UTIA Precision Beef Farm,
Johnson Research and Teaching Unit
Venue
University of Tennessee Conference Center
600 Henley Street, Knoxville, TN 37902, USA
Parking at Locust Street Garage
540 Locus St SW, Knoxville, TN 37902, USA
For participants not staying at the Marriot Knoxville Downtown, nor using their valet parking
Lodging
Marriott Knoxville, Downtown
525 Henley Street, Knoxville, TN 37902, USA
Contact
Dr. Robert Burns – rburns@utk.edu
Dr. Yang Zhao – yzhao@utk.edu
Organized by
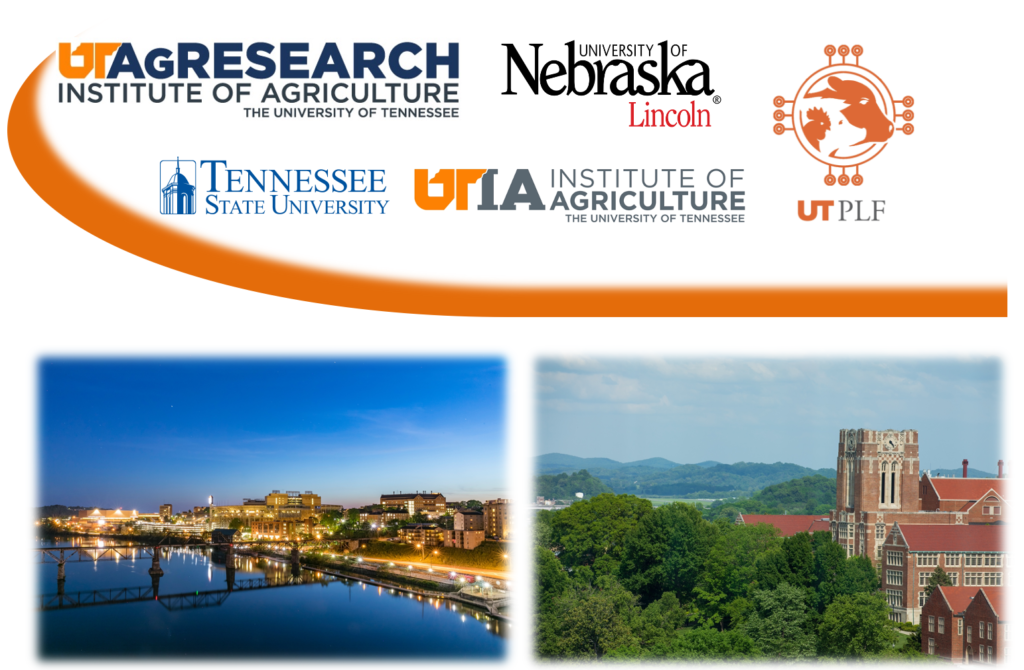